2024. 11. 13. 23:29ใArtificialIntelligence/ECCV2024
ECCV 2024. 10. 01. Tuesday
Oral 2B: Recognition
1) Generative Models
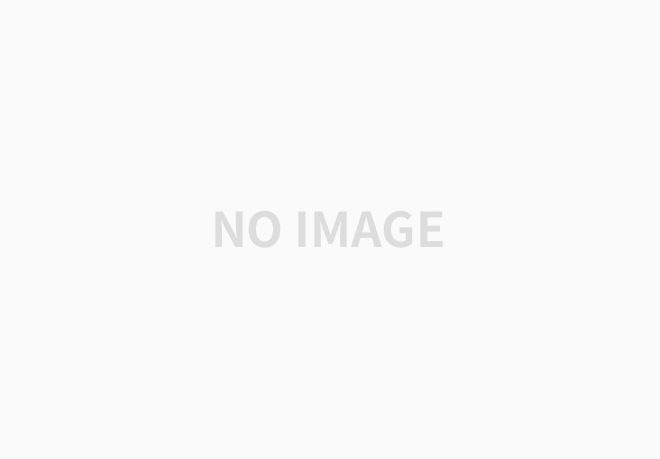
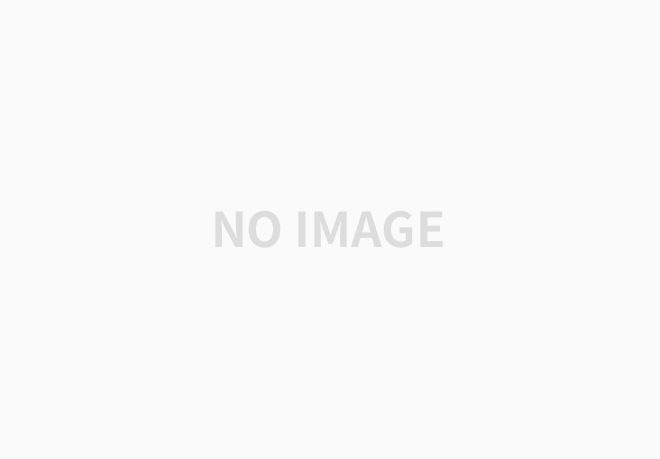
Gold Room์์ ์์ฑ๋ชจ๋ธ ์ค๋ด ์ธ์ ์ด ์์๋ค.
์์ฒญ์์ฒญ์์ฒญ ๋์ ํ์ด์๋ค ๐ฒ
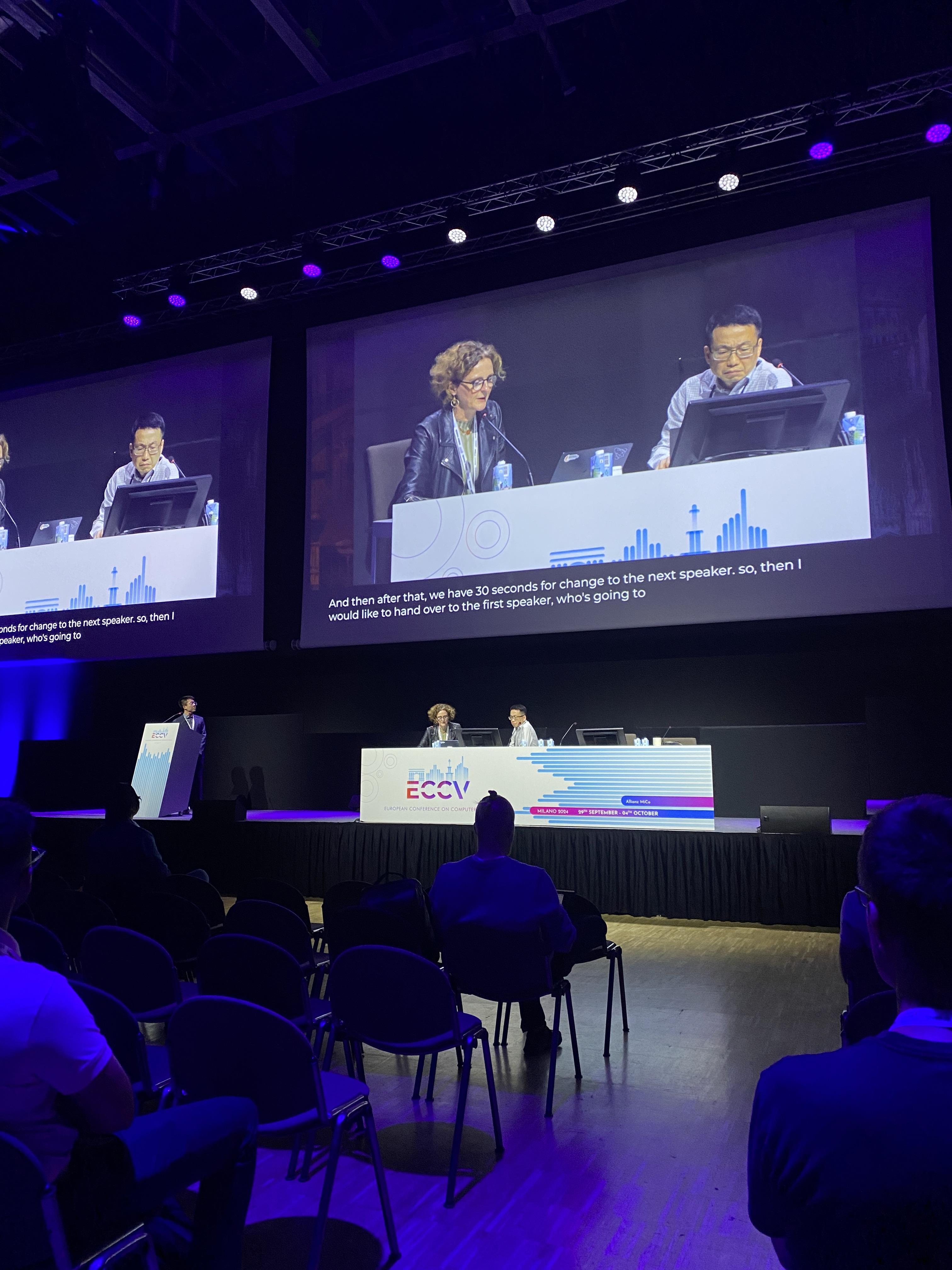
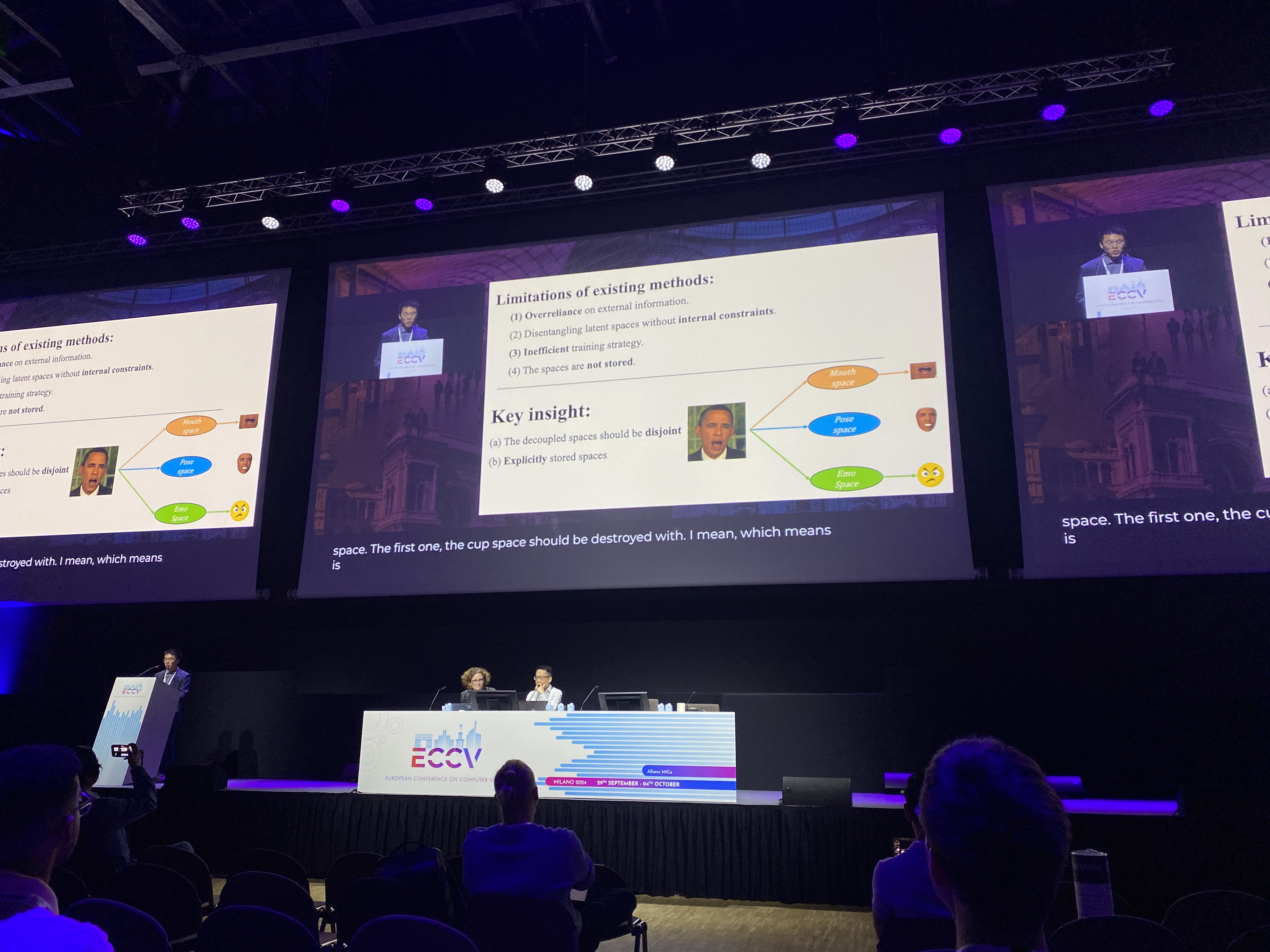
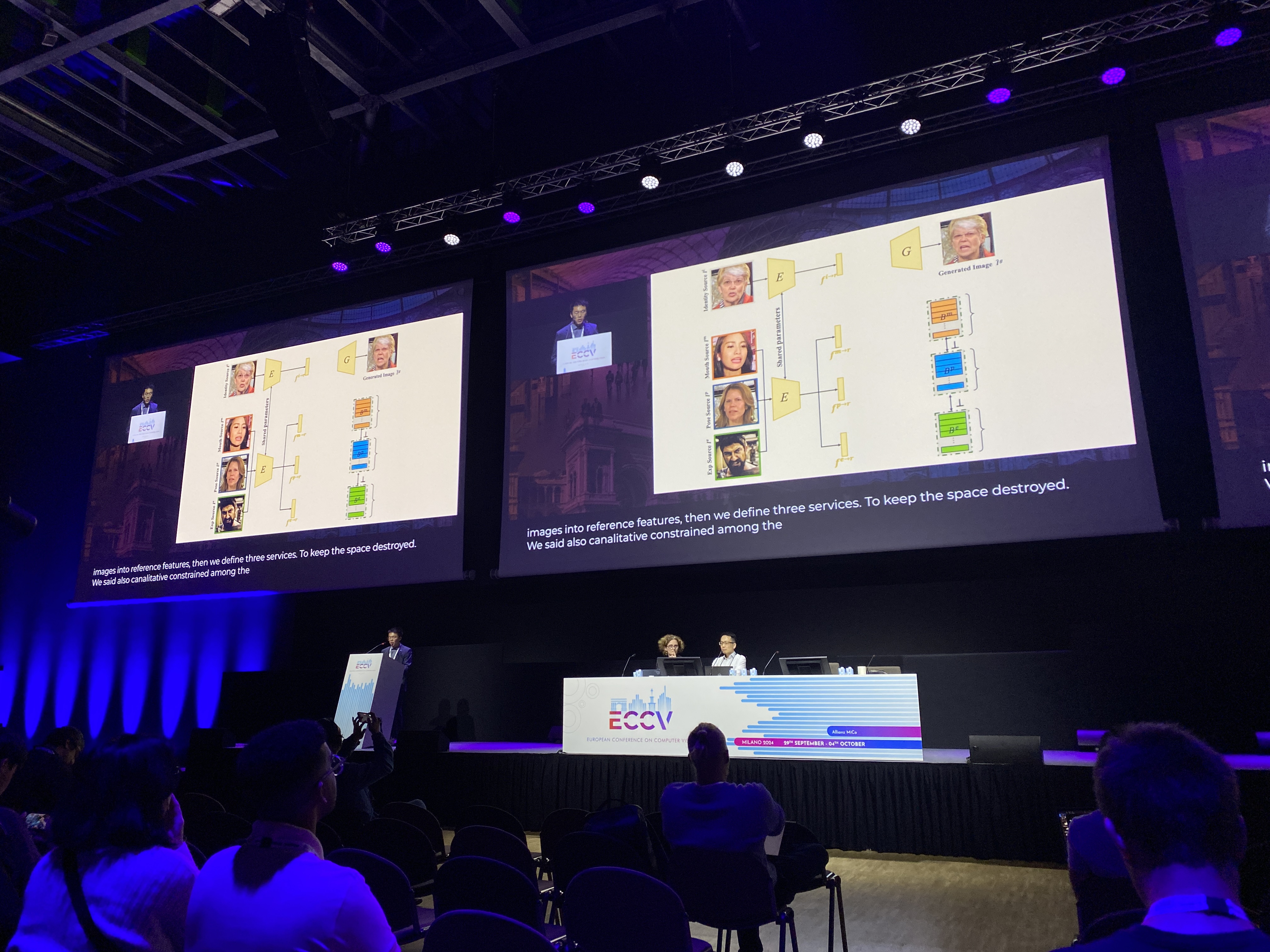
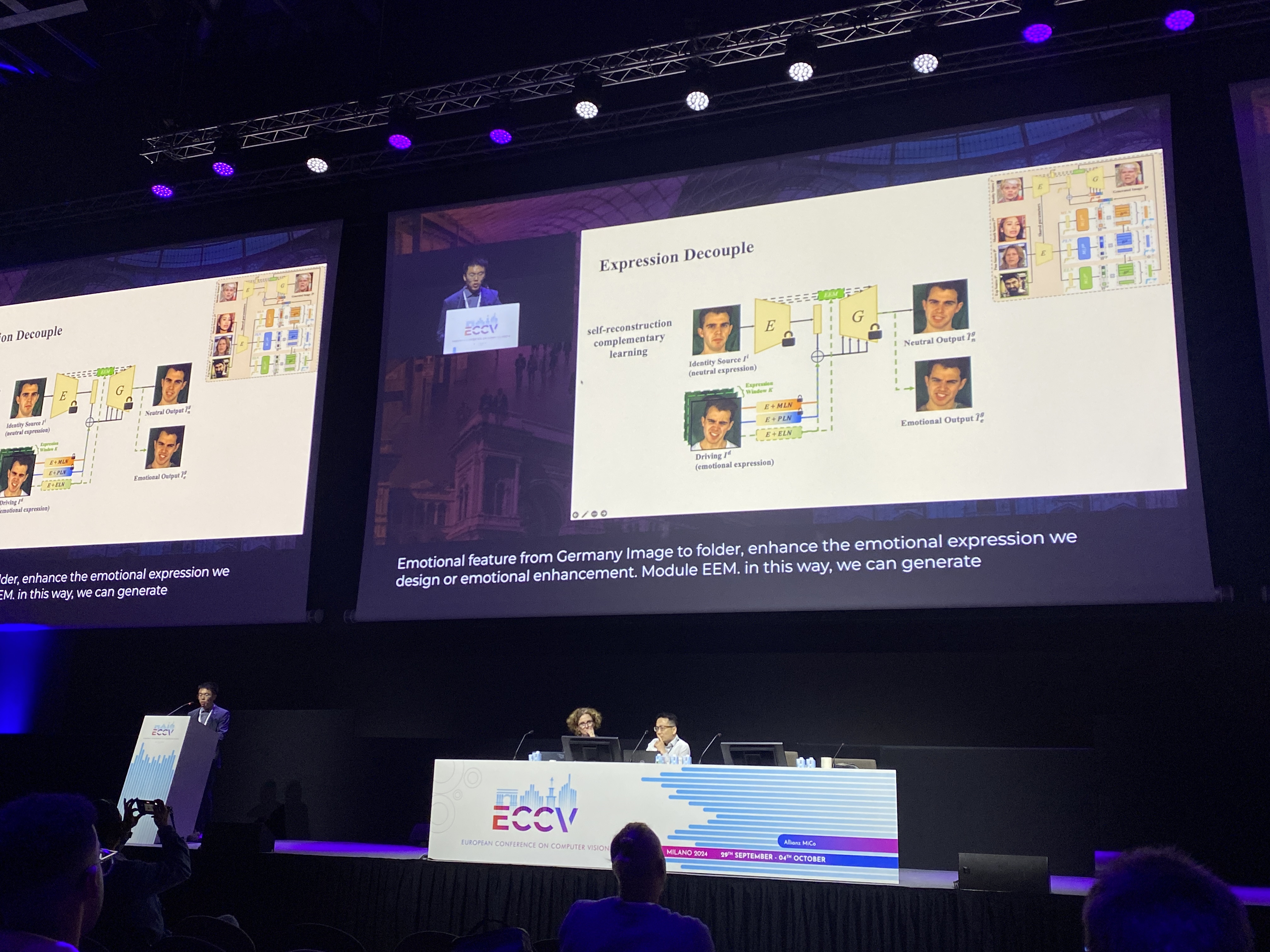
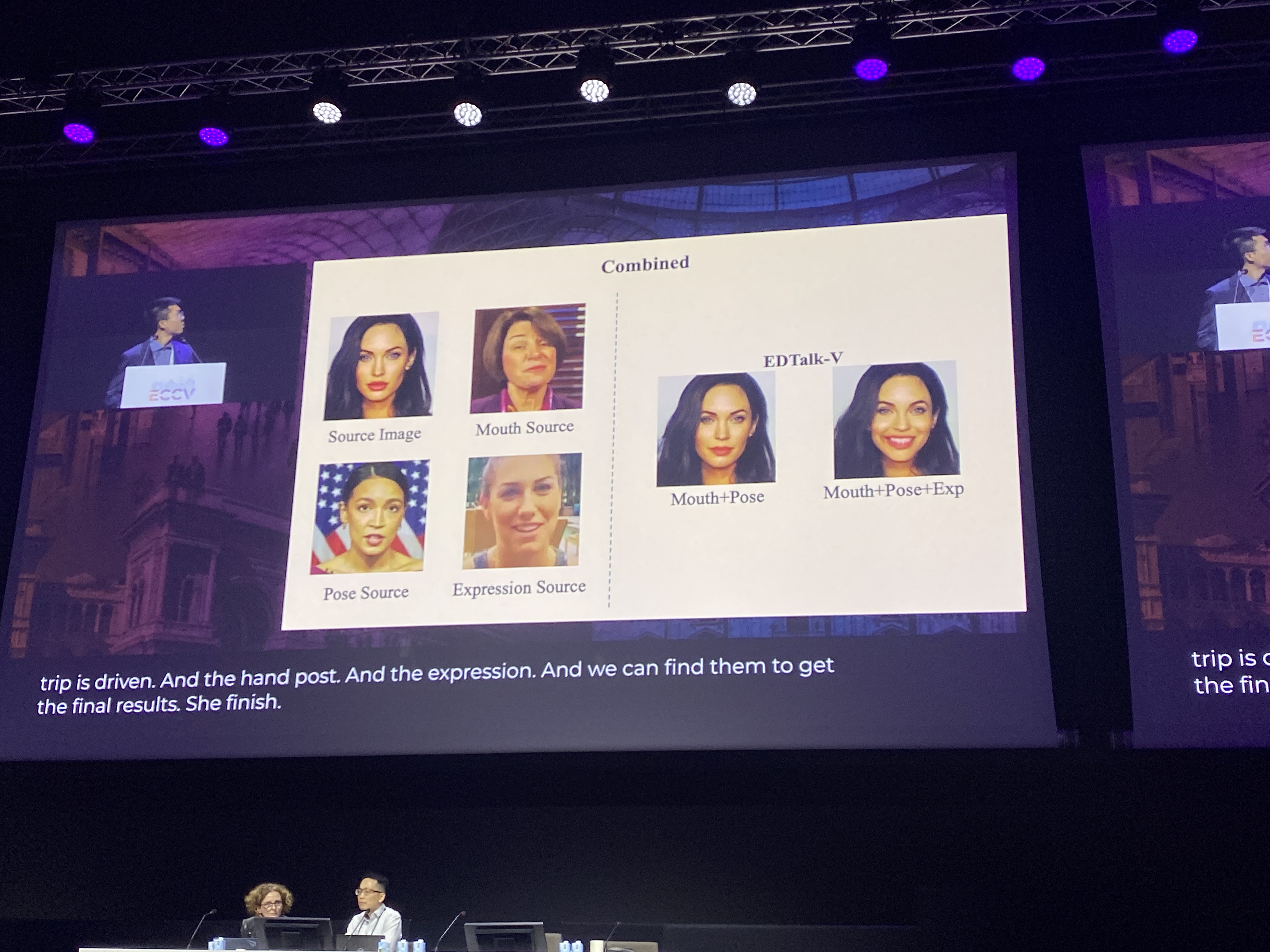
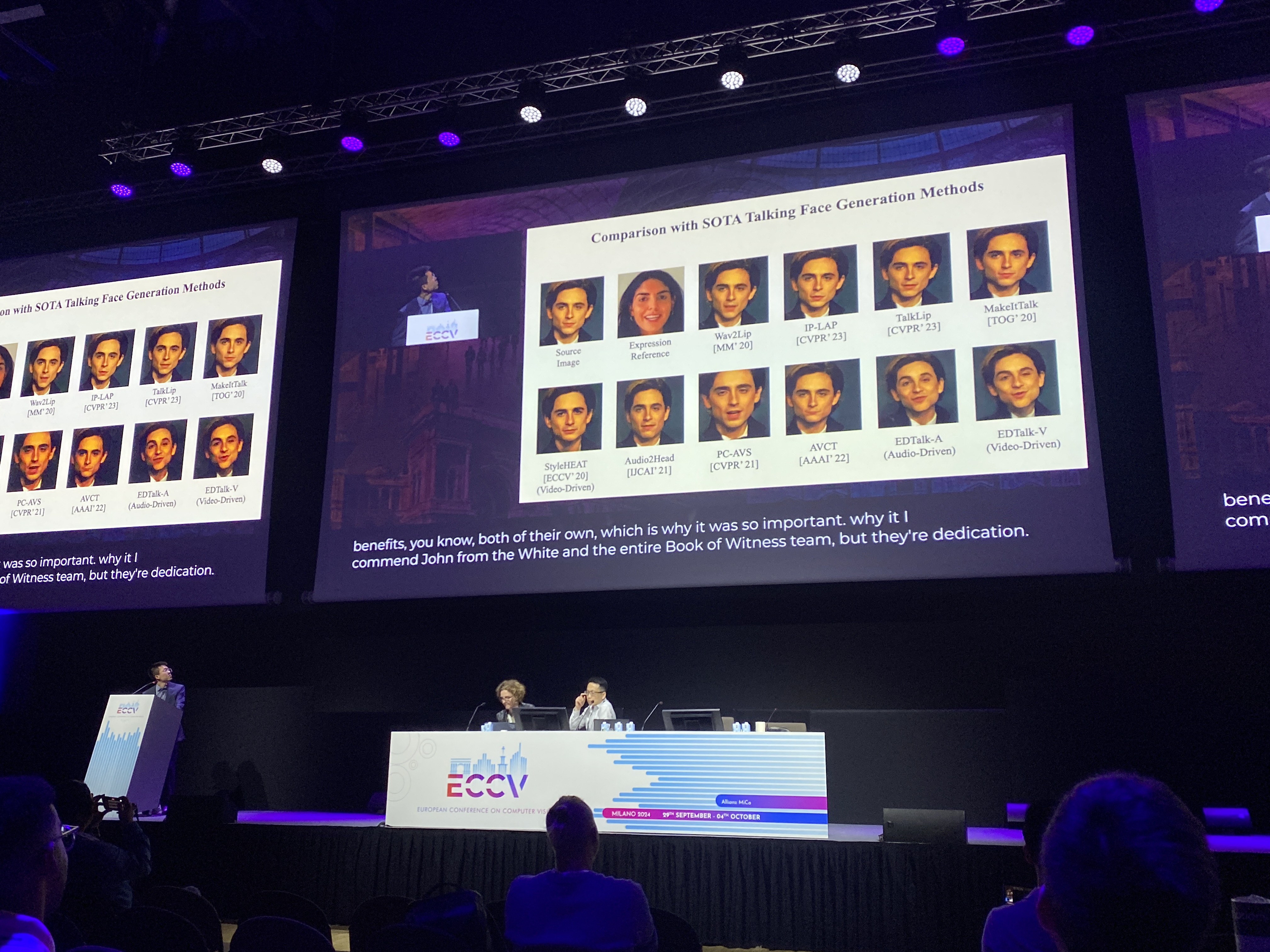
๋ฐํ ๋ฃ๋ค๊ฐ ํฐ๋ชจ์ ์ฌ๋ผ๋ฉ๊ฐ ๋ฑ์ฅํด์ ๋๋ฌด ๋ฐ๊ฐ์ ๋ค.
๊ทธ๋ฐ๋ฐ ์ฐ์์ธ ์ฌ์ง๋ค์ ์ ๋ ๊ฒ ๋ง ์ฌ์ฉํด๋ ๊ด์ฐฎ์๊ฑด๊ฐ..? ์ด๋ฐ ์๊ฐ๋ ๋ค์๋ค.
AI ๋ชจ๋ธ์ ๋๋ฆด ๋, ์ด์๊ถ์ ๋ณ๊ฐ์ ๋ฌธ์ ์ธ๊ฑด๊ฐ .?? ๋ฌดํผ ..
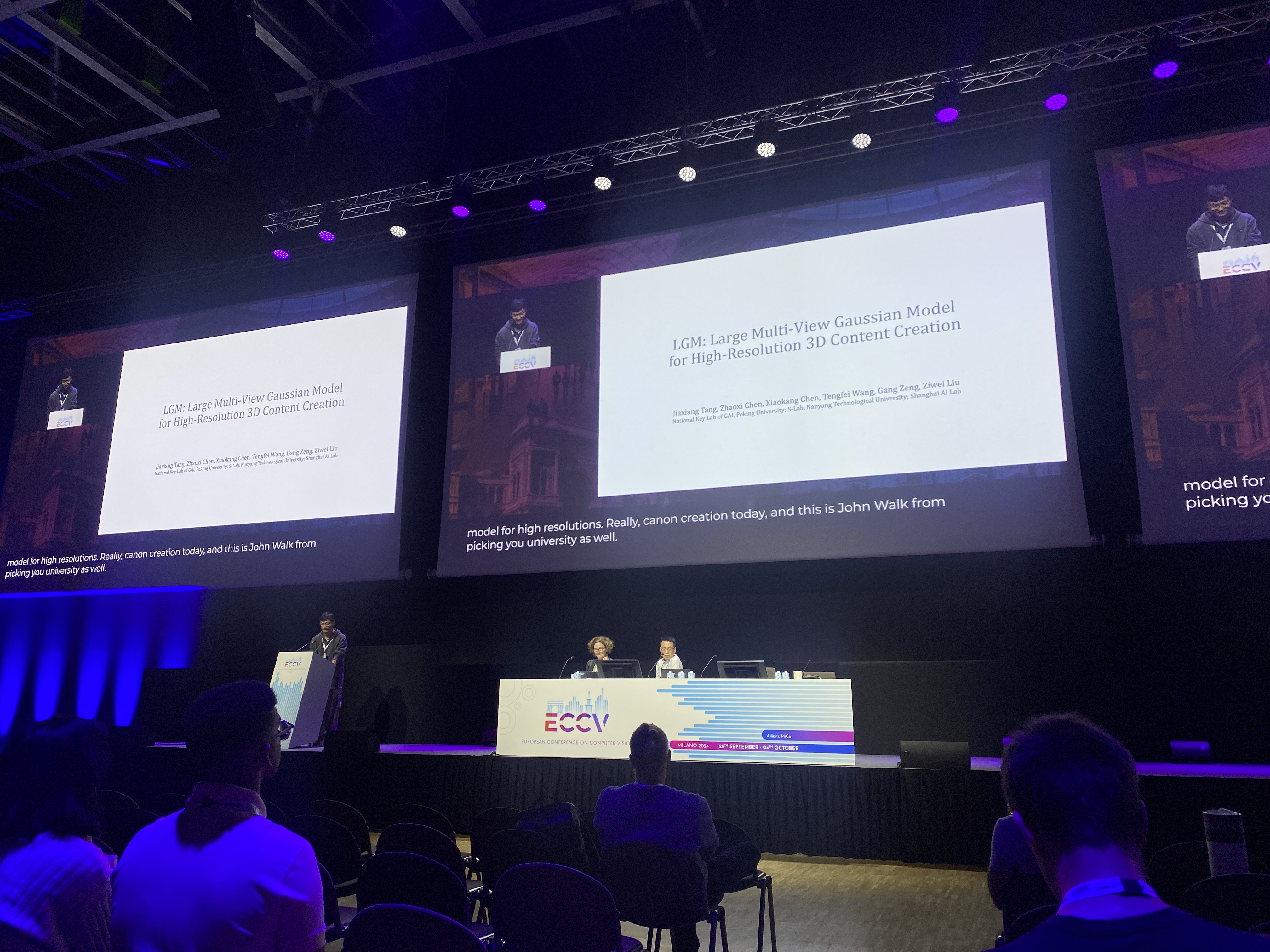
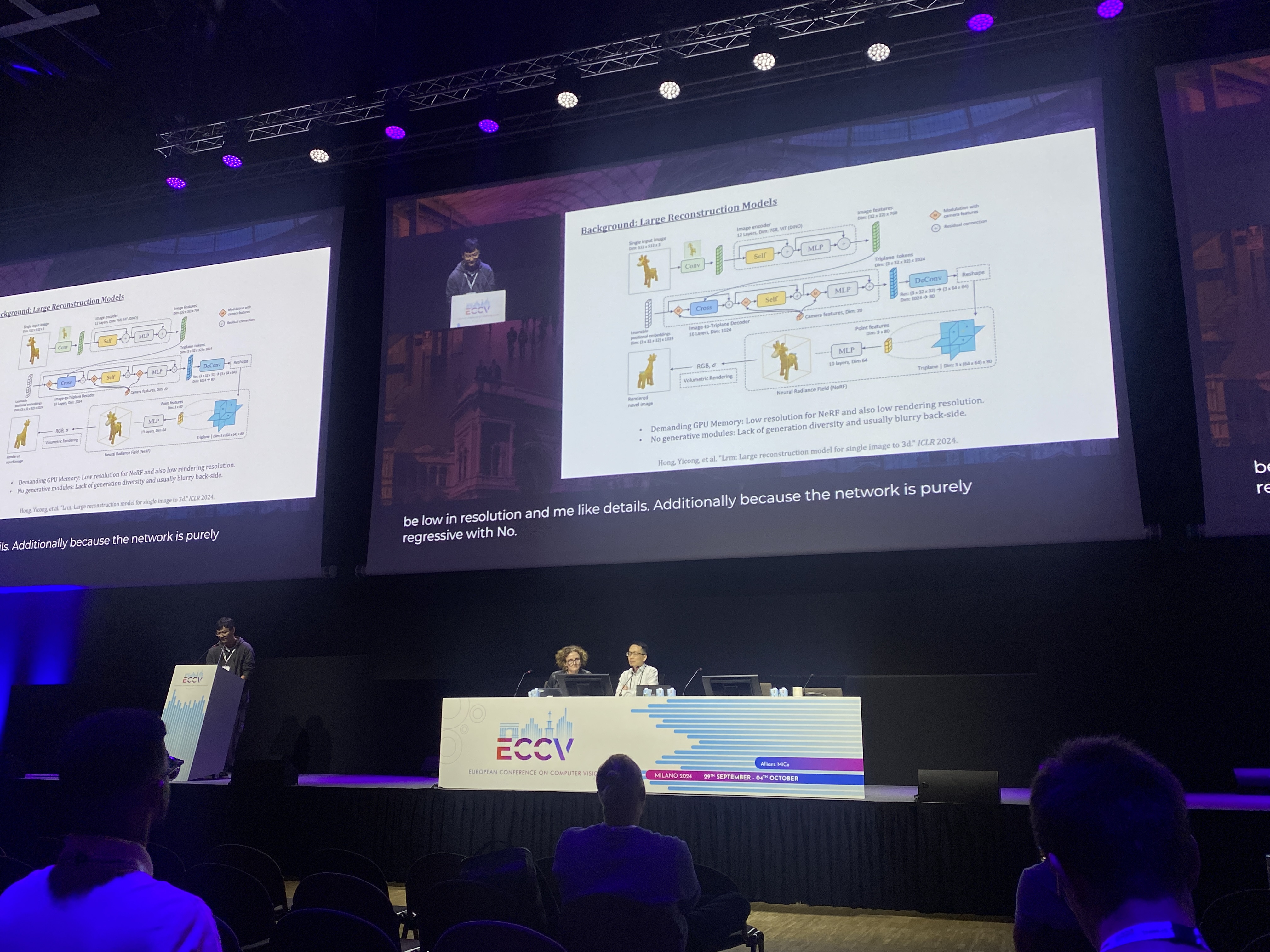
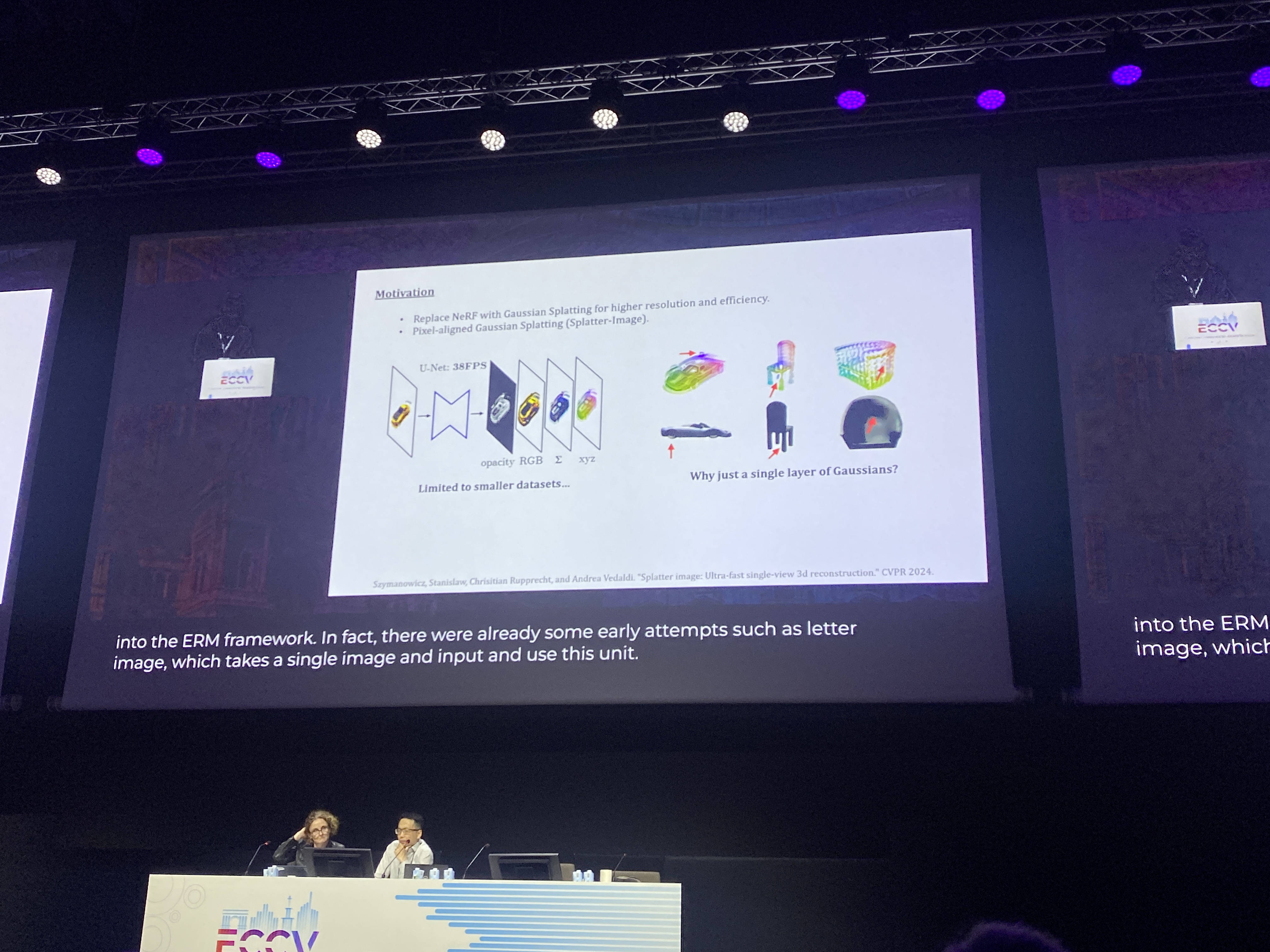
์๋ฌด๋๋ ์๊ฐ์ ์ผ๋ก ๋ณด์ฌ์ง๋๊ฒ ๋ง์์, ์ฌ๋ฏธ์๊ฒ ๋ค์์ง๋ง,
๊ธฐ์ ์์ฒด์ ์๋ฏธ๊ฐ ์๋ค๊ณ ๋๋ผ์ง๋ ๋ชปํ๋ค.
2) Recognition
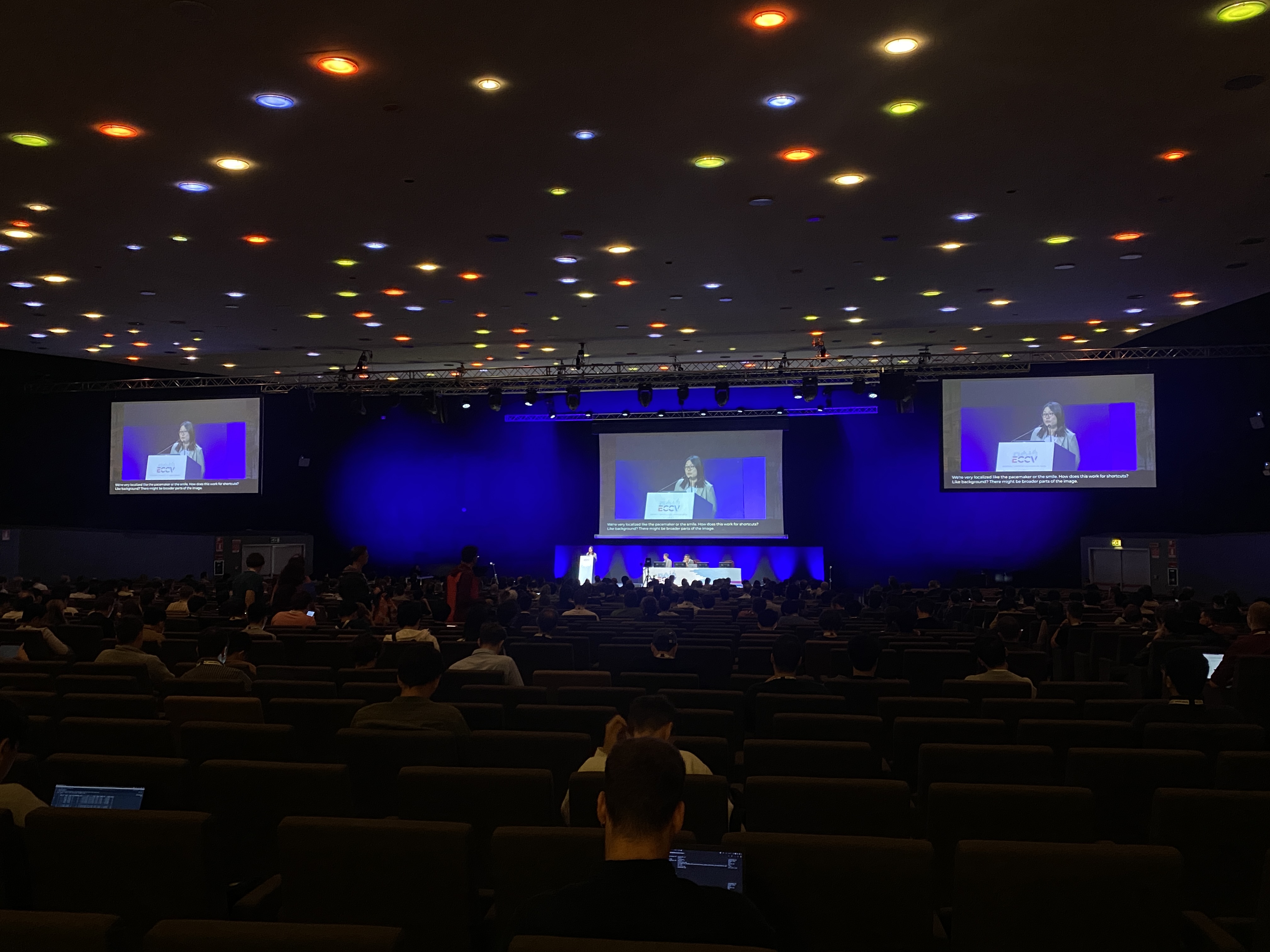
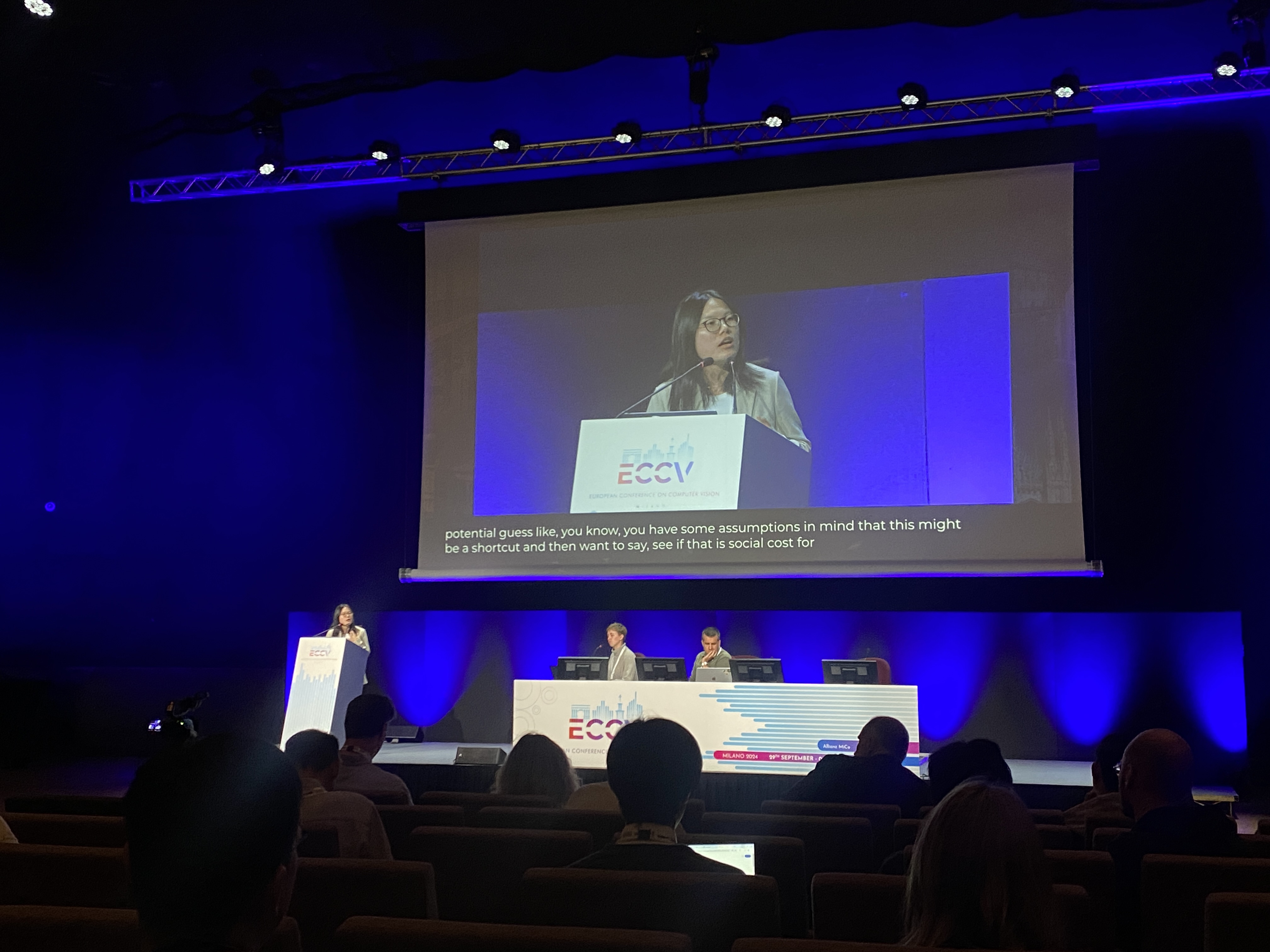
# Google Mobile-Net
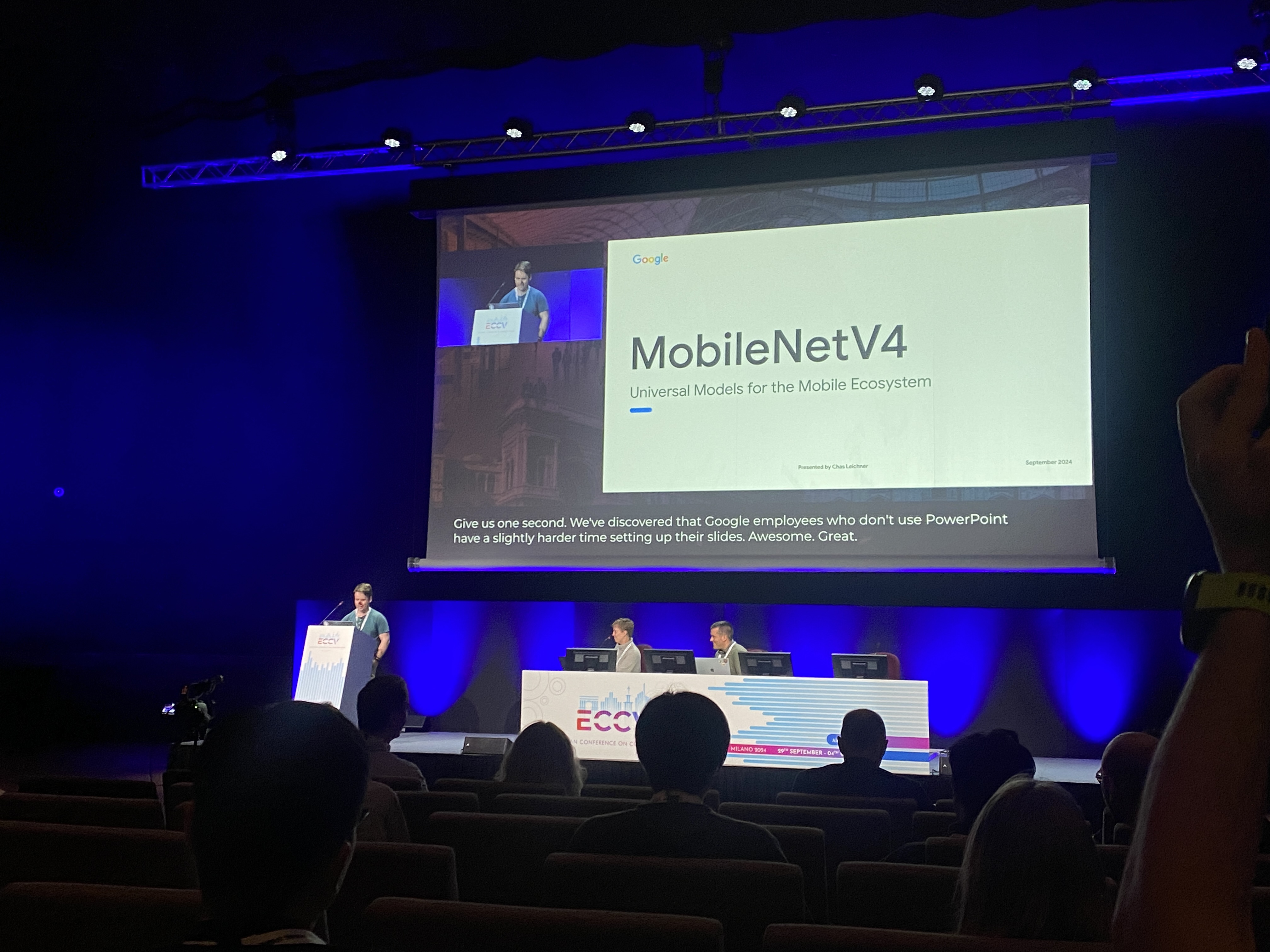
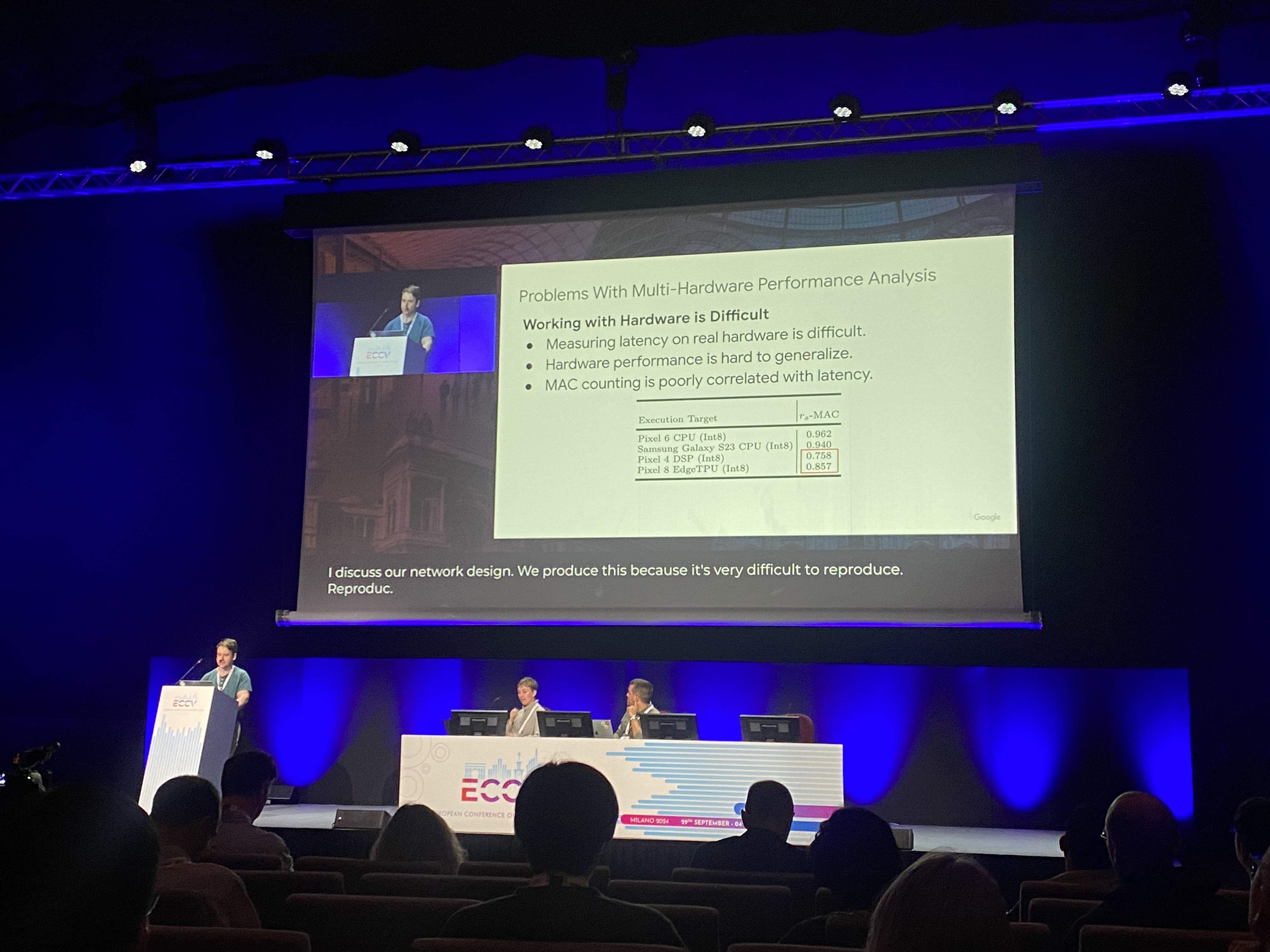
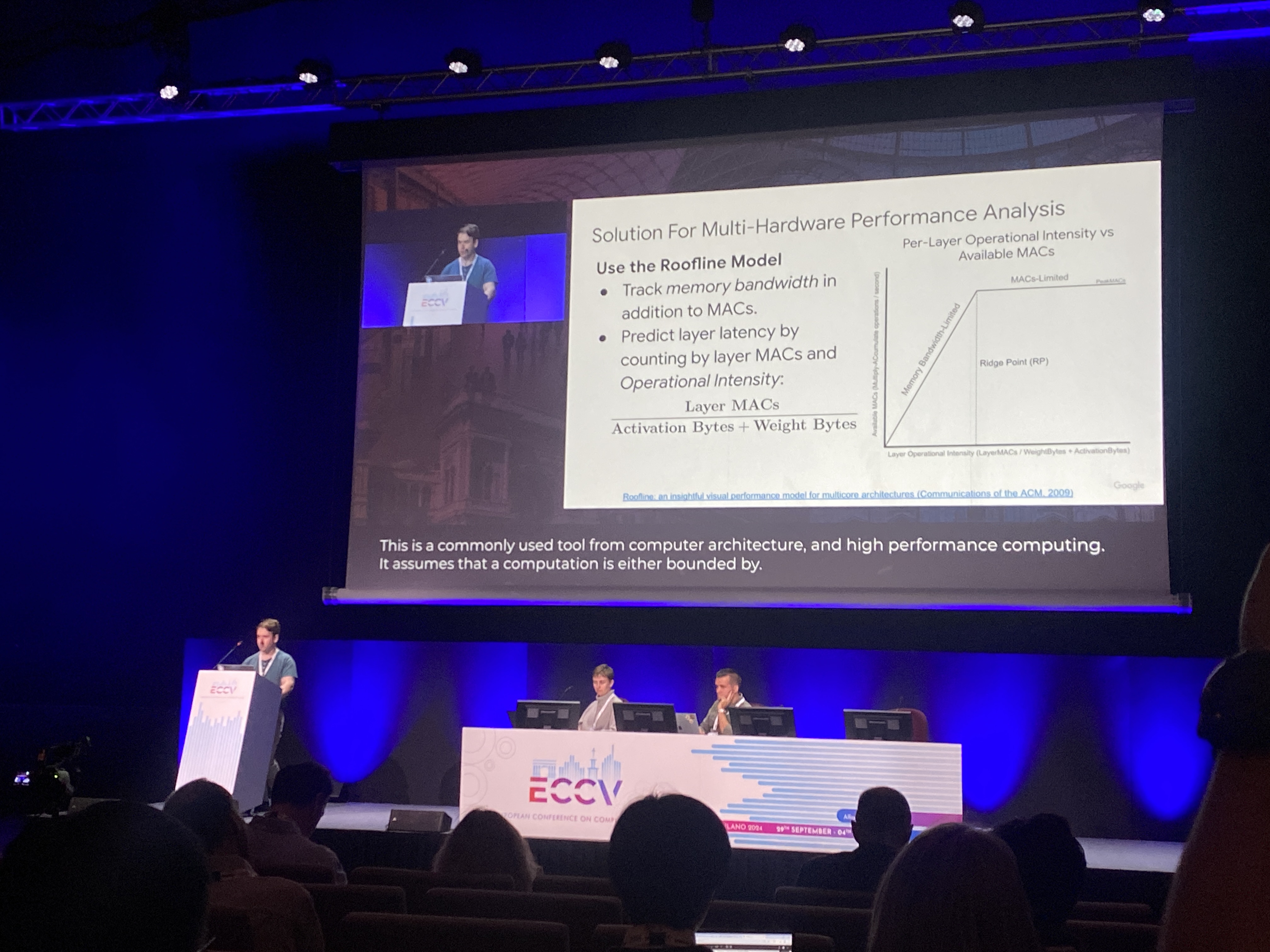
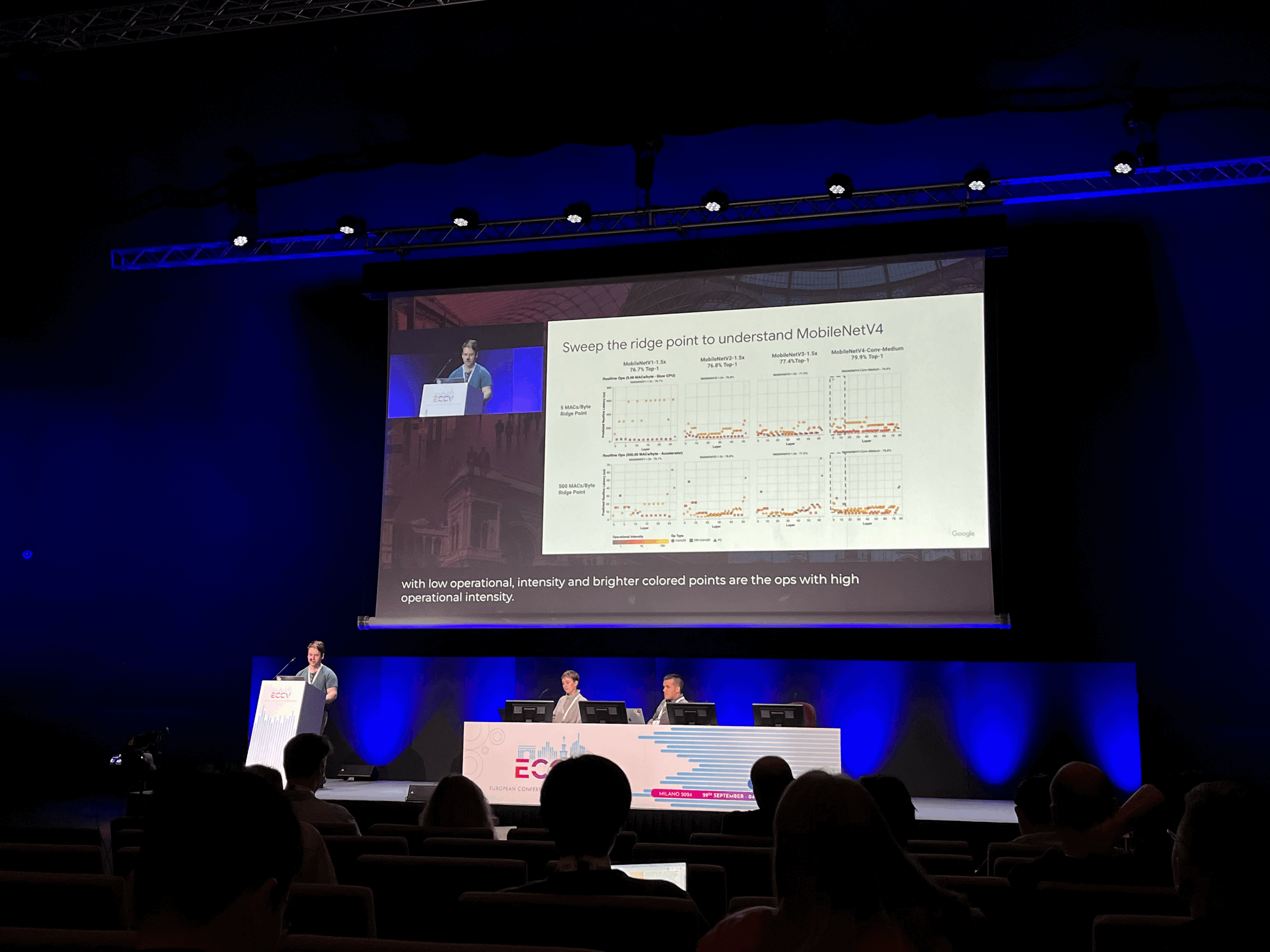
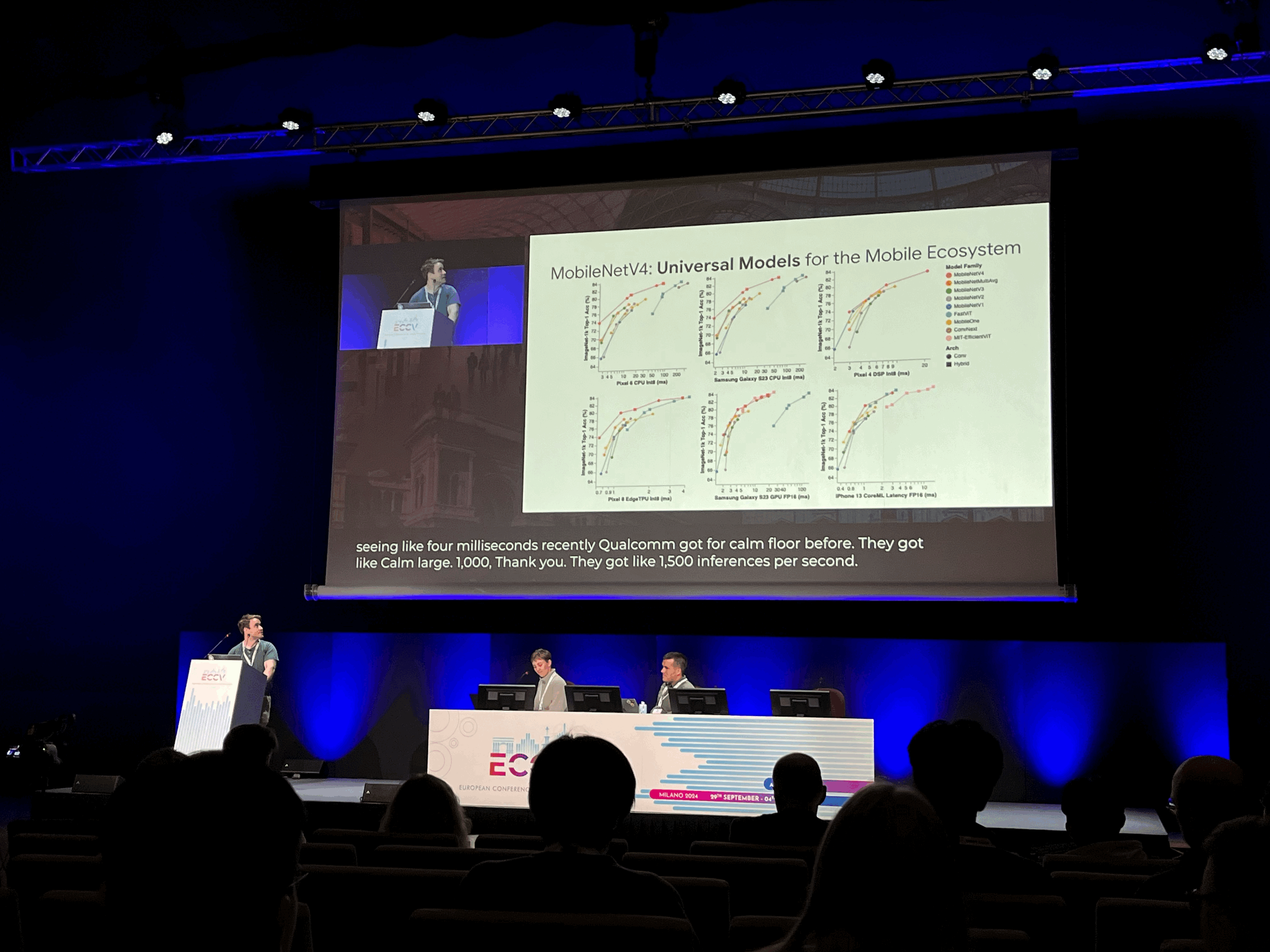
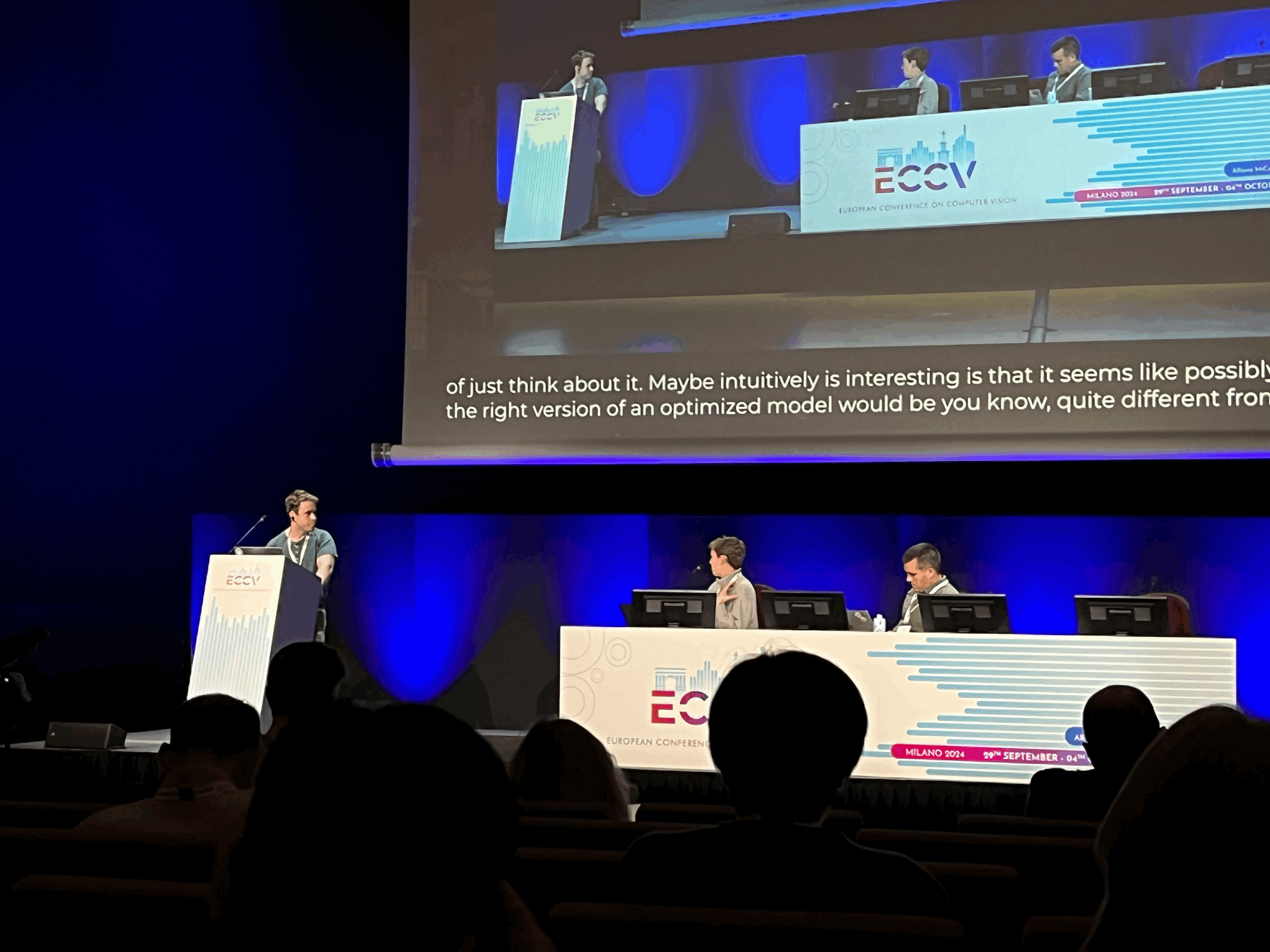
MobileNetV4: Universal Models for the Mobile Ecosystem - Google
https://eccv.ecva.net/virtual/2024/oral/482
https://www.ecva.net/papers/eccv_2024/papers_ECCV/papers/05647.pdf
+ ํ๋์จ์ด์ ๋ํ ๊ณ ๋ฏผ์ด ๋ด๊ฒจ์ ์ฌ๋ฏธ์์๋ค. (์ด๋ป๊ฒ ์ฑ๋ฅ์ ๋ถ์ํ ์ง์ ๋ํ ๋ฐฉ์๋ค)
Abstract.
We present the latest generation of MobileNets: MobileNetV4 (MNv4). They feature universally-efficient architecture designs for mobile devices. We introduce the Universal Inverted Bottleneck (UIB) search block, a unified and flexible structure that merges Inverted Bottleneck (IB), ConvNext, Feed Forward Network (FFN), and a novel Extra Depthwise (ExtraDW) variant. Alongside UIB, we present Mobile MQA, an attention block for mobile accelerators, delivering a significant 39% speedup. An optimized neural architecture search (NAS) recipe is also introduced which improves MNv4 search effectiveness.
The integration of UIB, Mobile MQA and the refined NAS recipe results in a new suite of MNv4 models that are mostly Pareto optimal across mobile CPUs, DSPs, GPUs, as well as accelerators like Apple Neural Engine and Google Pixel EdgeTPU. This performance uniformity is not found in any other models tested. We introduce performance modeling and analysis techniques to explain how this performance is achieved. Finally, to further boost accuracy, we introduce a novel distillation technique. Enhanced by this technique, our MNv4-Hybrid-Large model delivers 87% ImageNet-1K accuracy, with a Pixel 8 EdgeTPU runtime of 3.8ms.
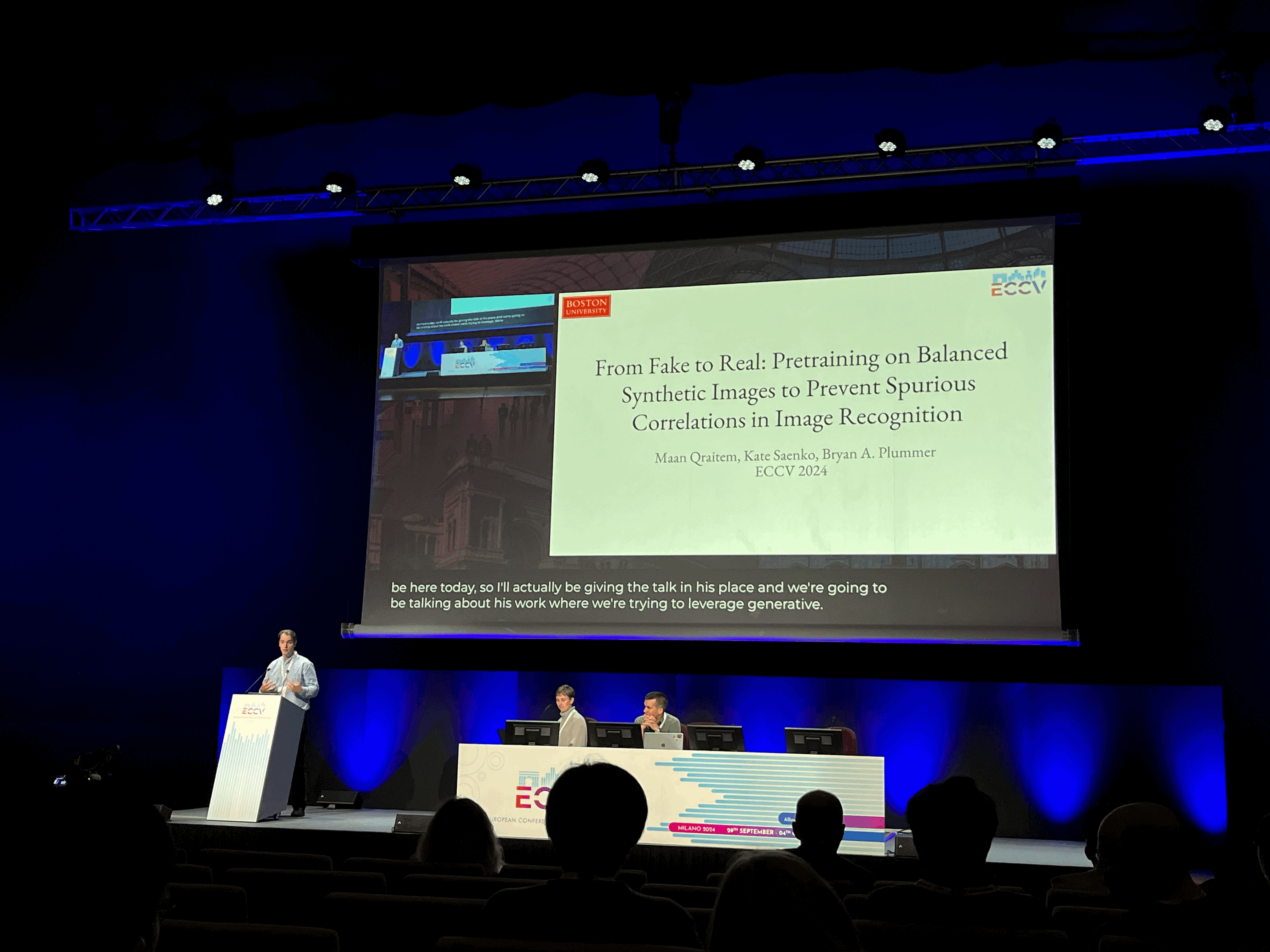
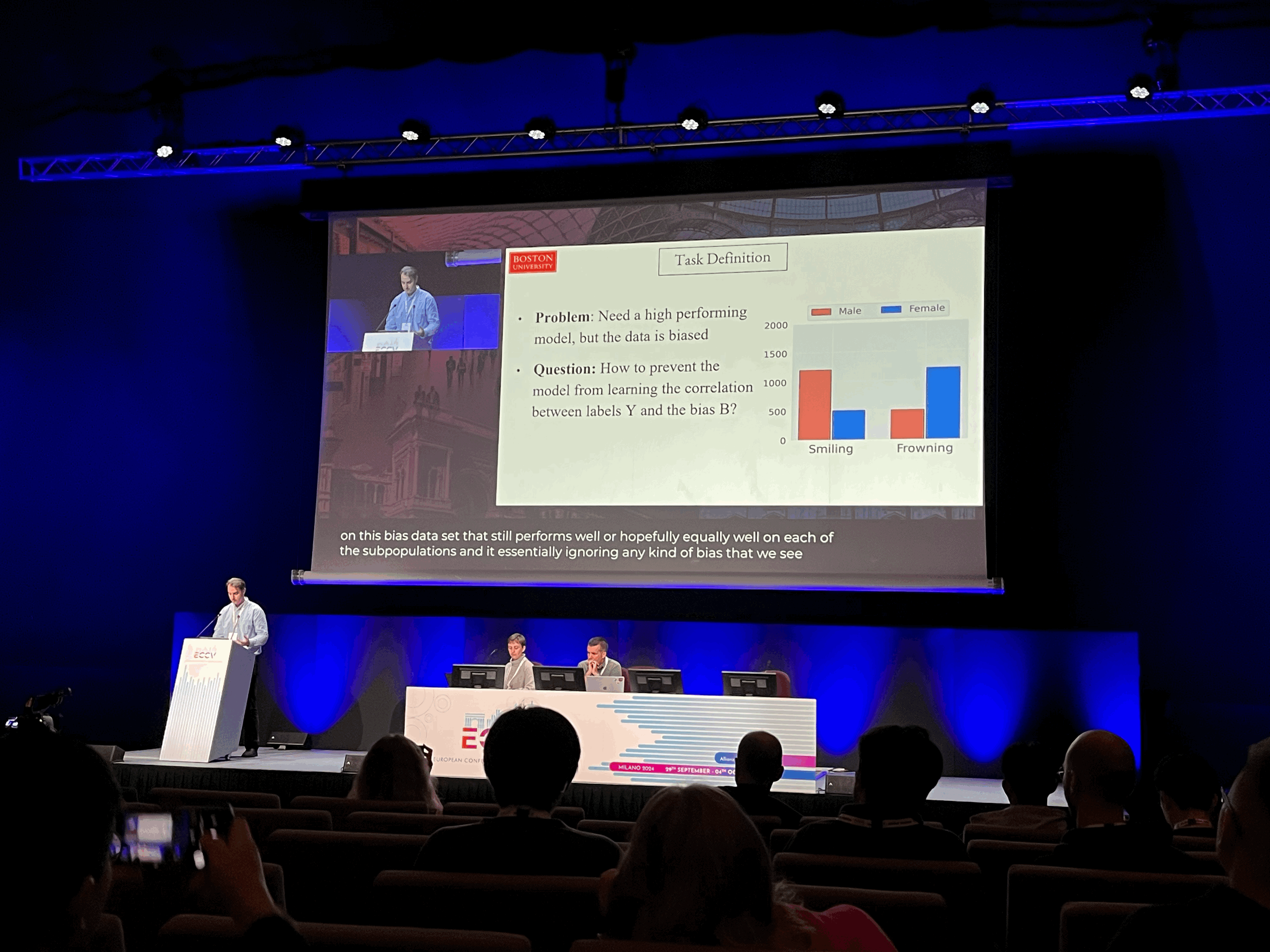
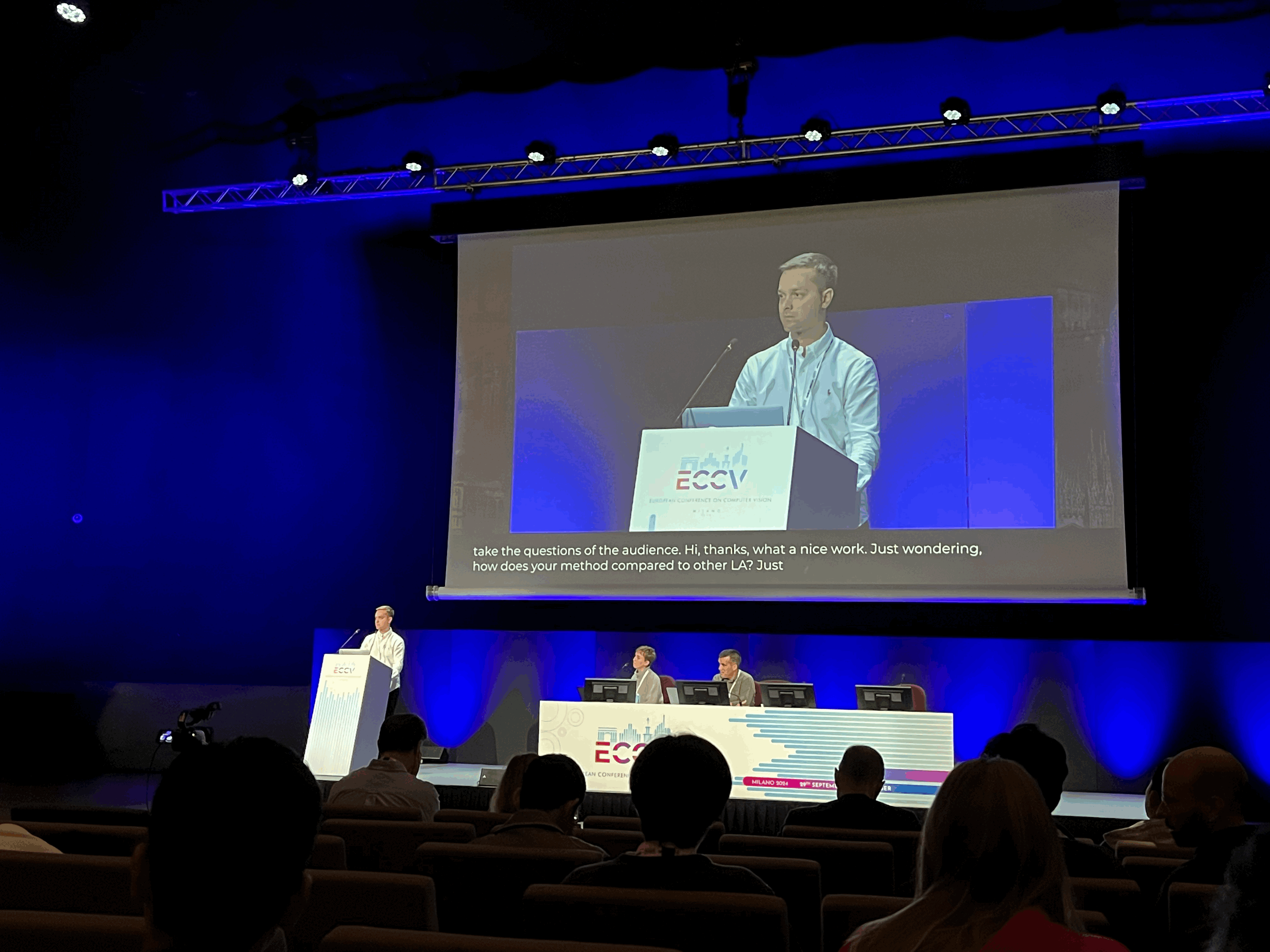
From Fake to Real: Pretraining on Balanced Synthetic Images to Prevent Spurious Correlations in Image Recognition
https://eccv.ecva.net/virtual/2024/oral/498
https://www.ecva.net/papers/eccv_2024/papers_ECCV/papers/07557.pdf
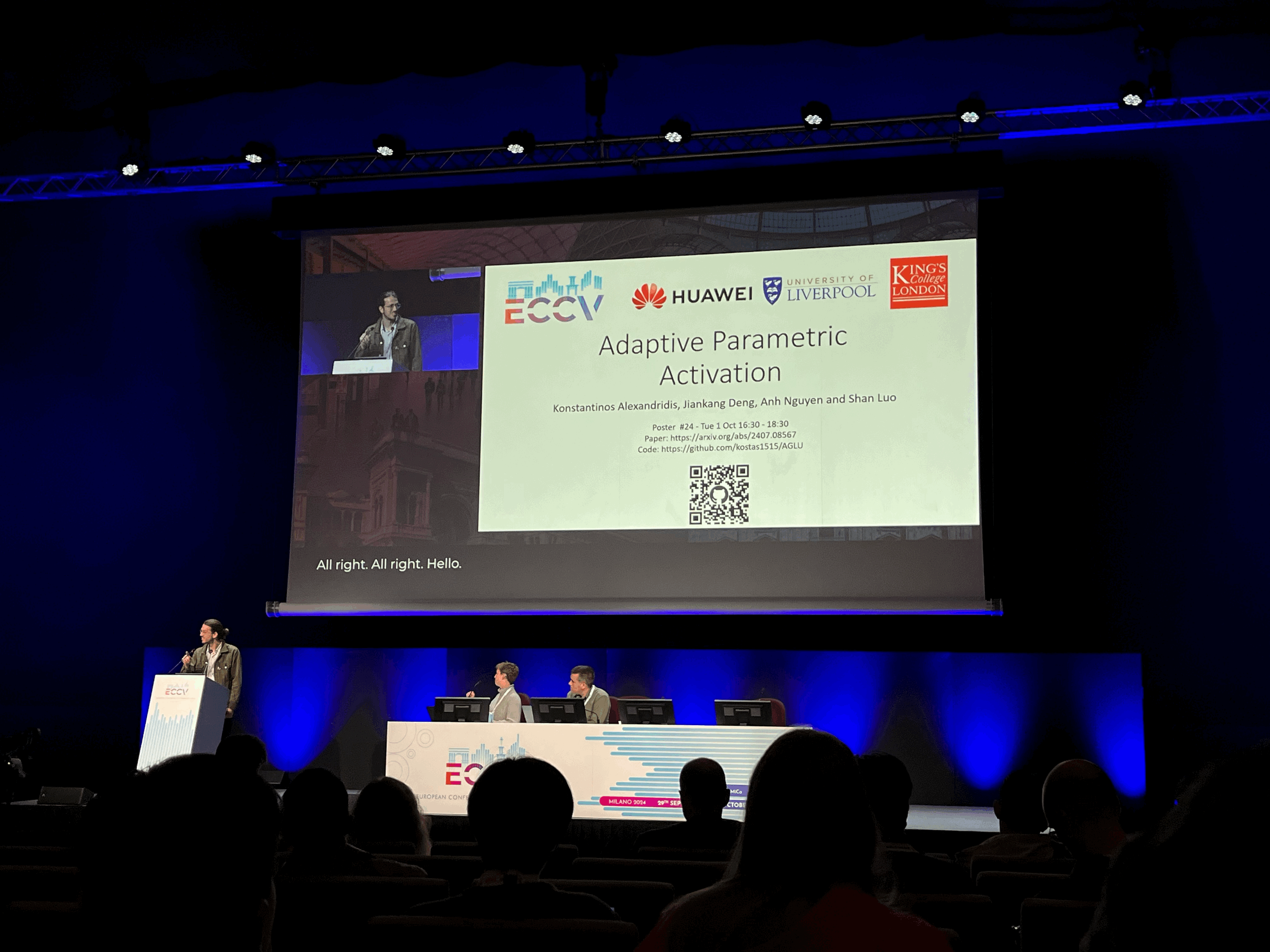
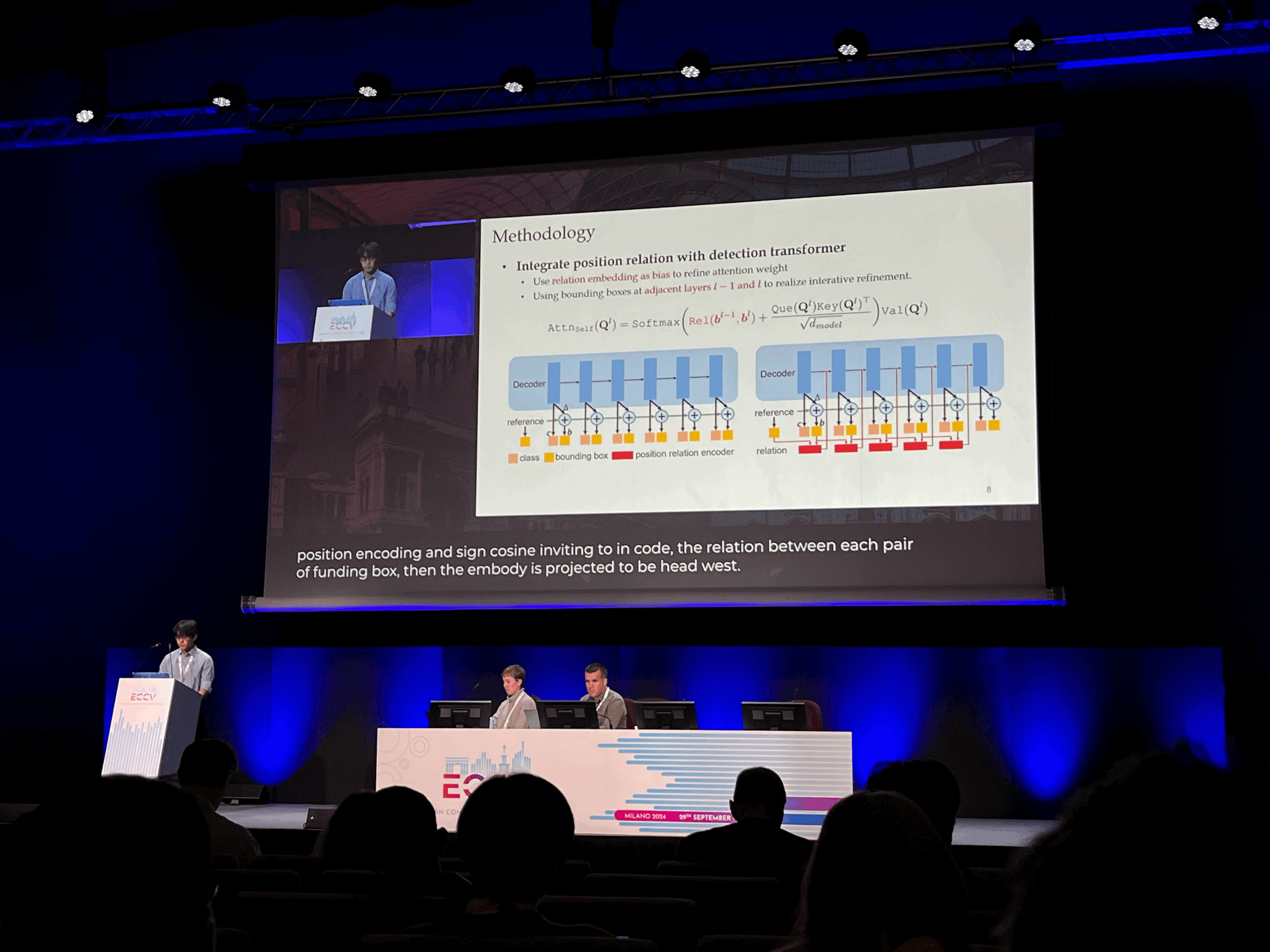
Relation DETR: Exploring Explicit Position Relation Prior for Object Detection
https://eccv.ecva.net/virtual/2024/oral/502
์ด ๋ค์์ผ๋ก ๋ฐํํ์ ์ฐ๊ตฌ ์ฃผ์ ๊ฐ ํฅ๋ฏธ๋ก์ ๋๋ฐ, ์์ ์ ์ 'Still Me' ๋ผ๊ณ ํ์ ์ ์งฑ๋ฉ์ก๋ค.
(๊ฐ์ ๋ถ์ด ๋ฐํ๋ฅผ ์ฐ๋ฌ์์ ํ์ จ๋ค.)
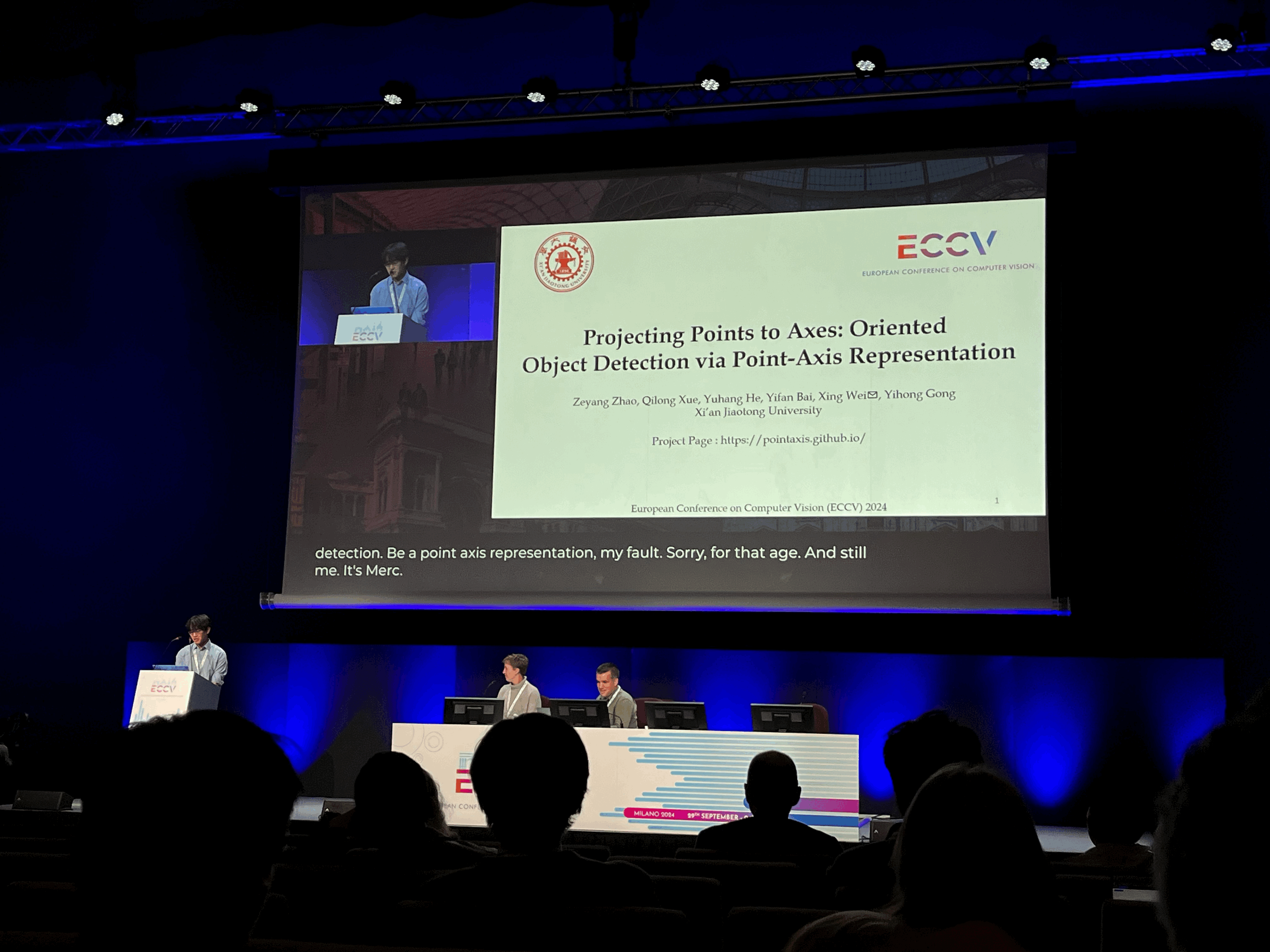
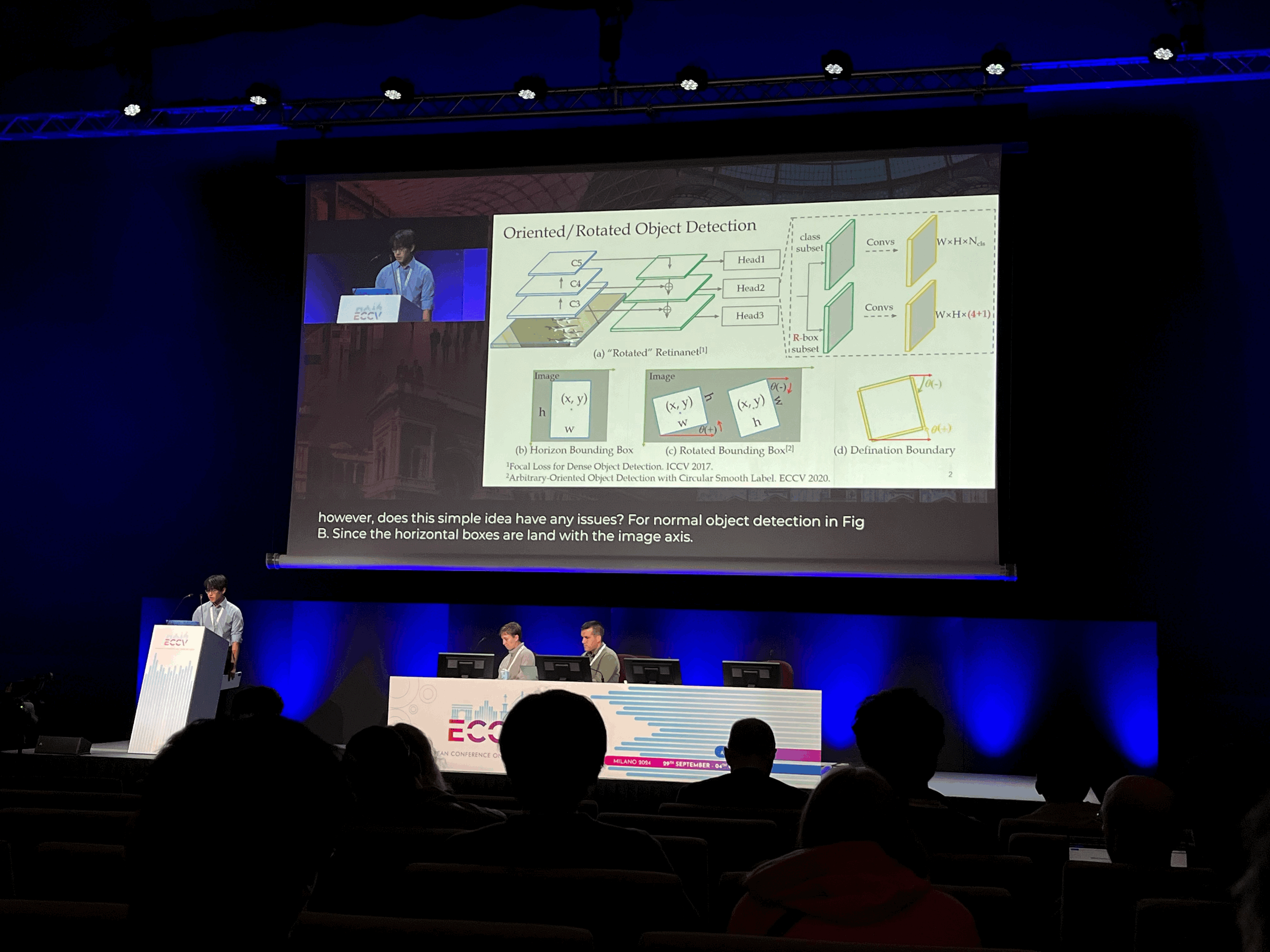
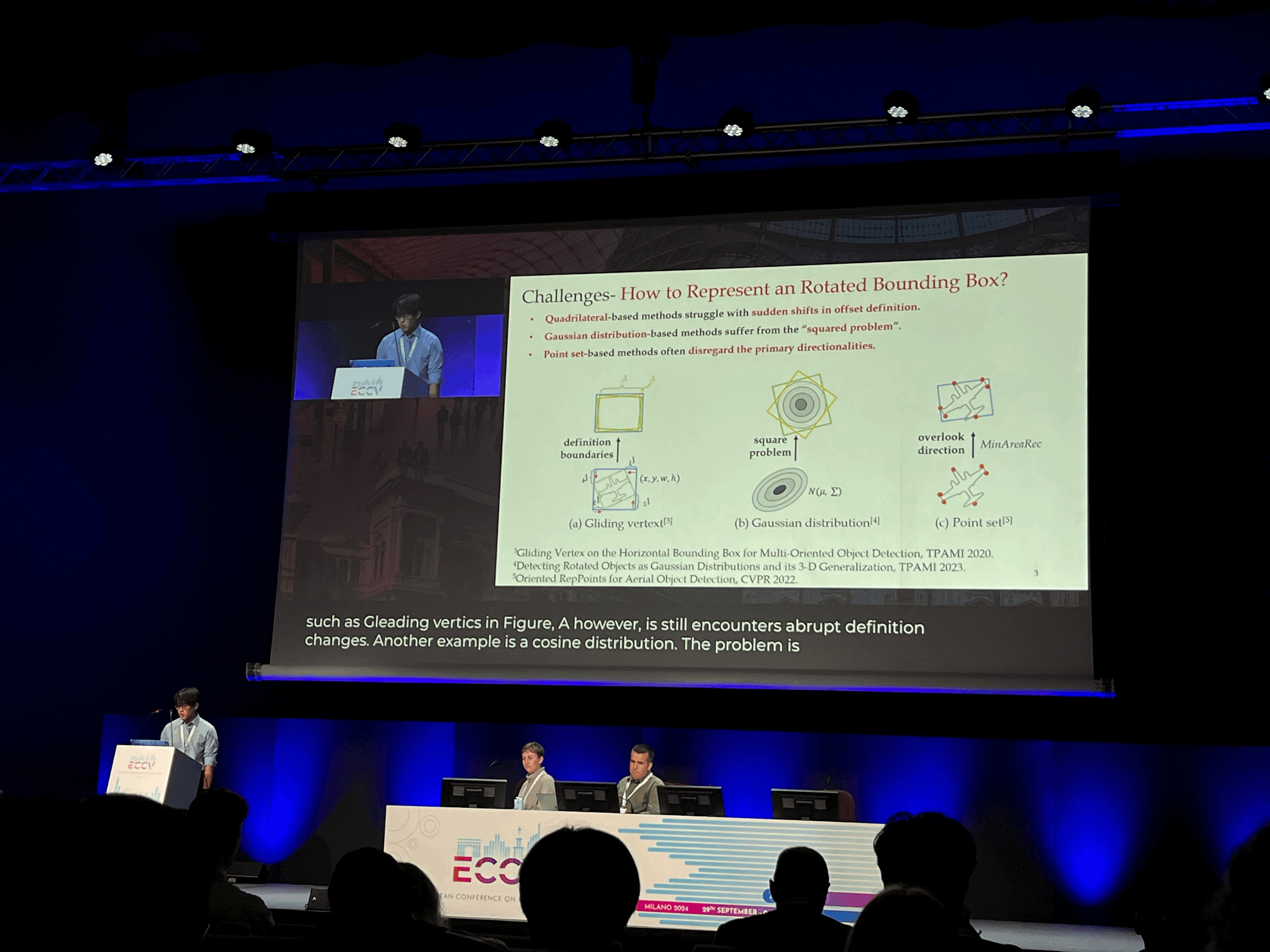
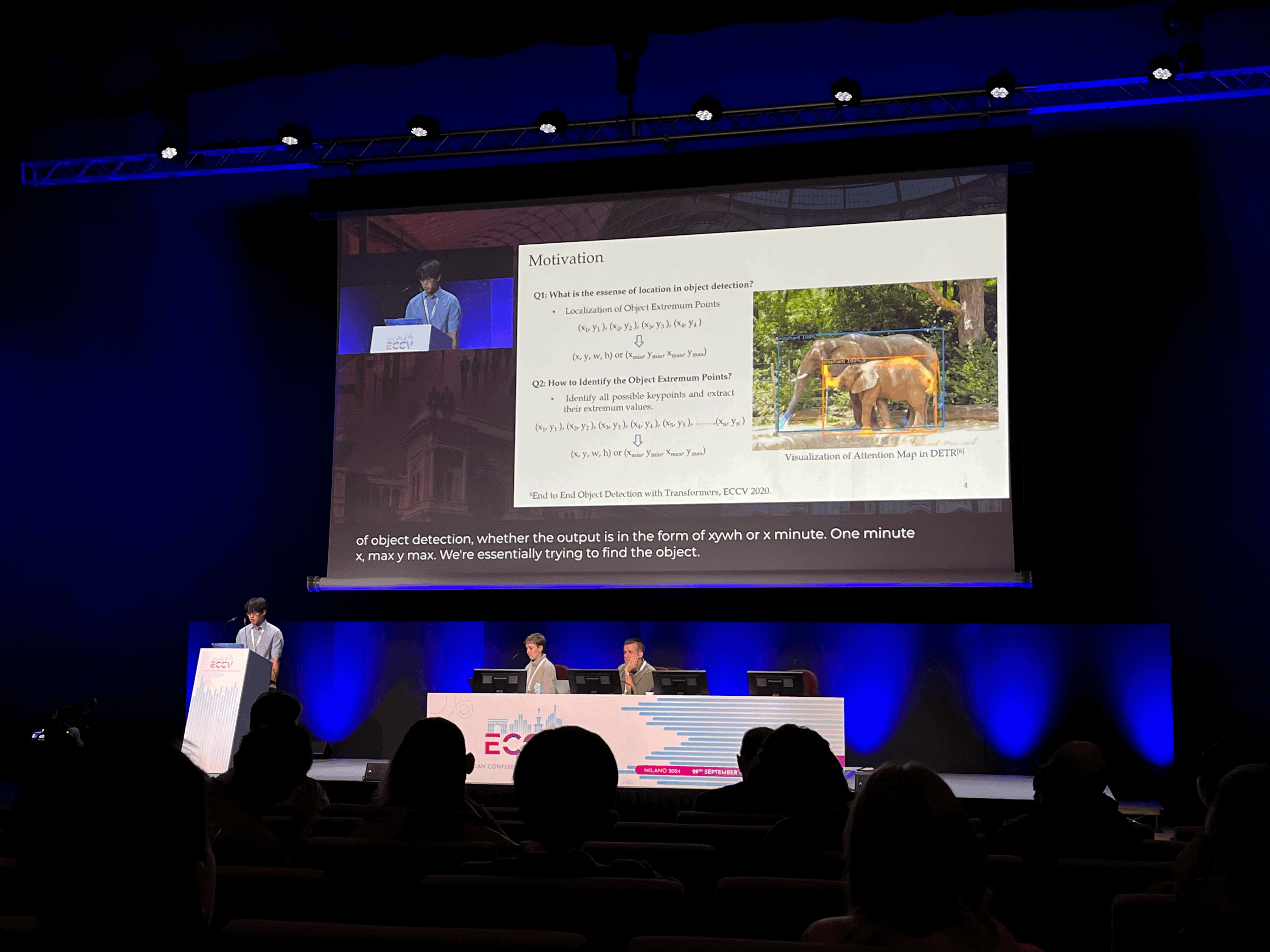
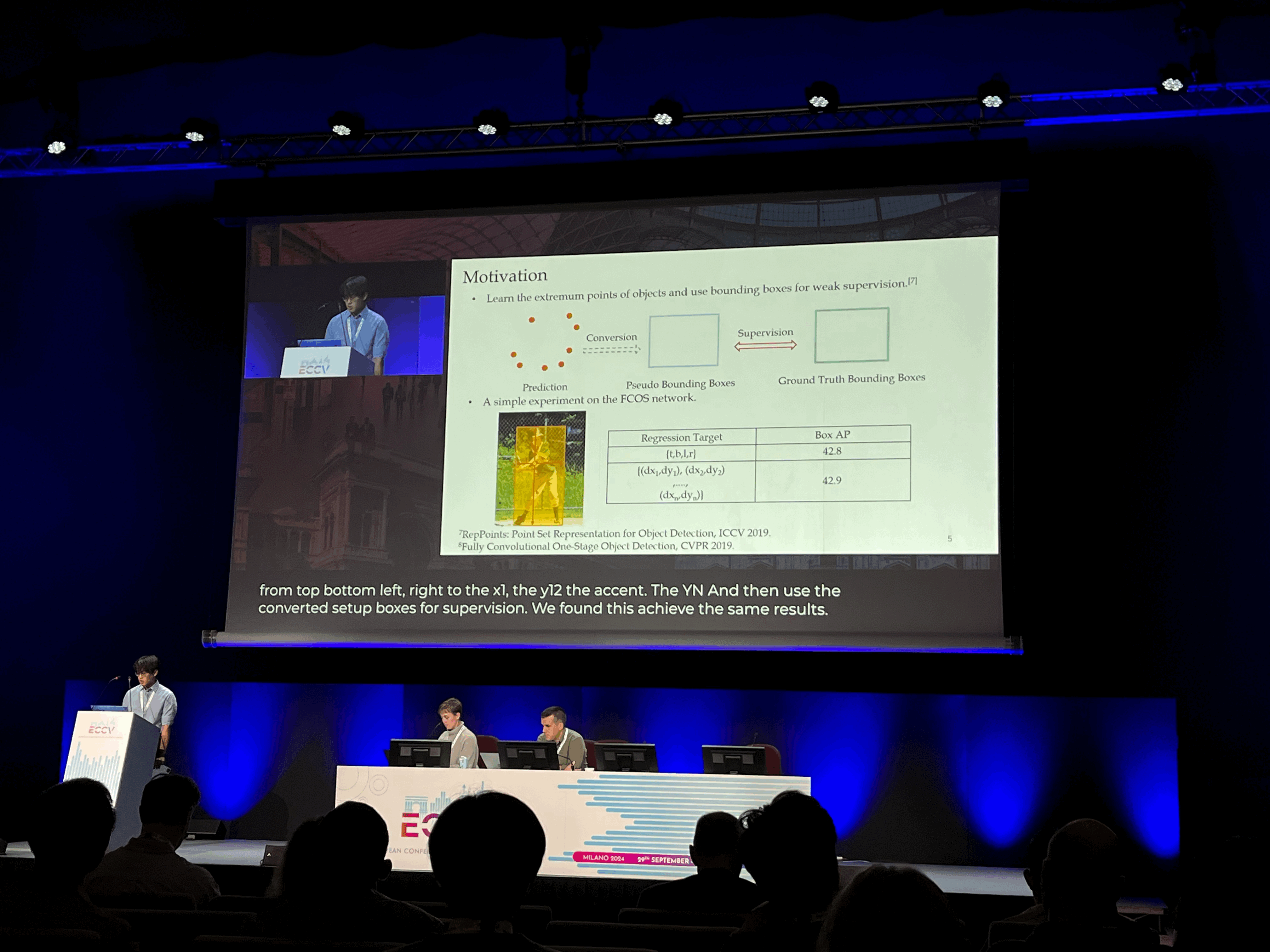
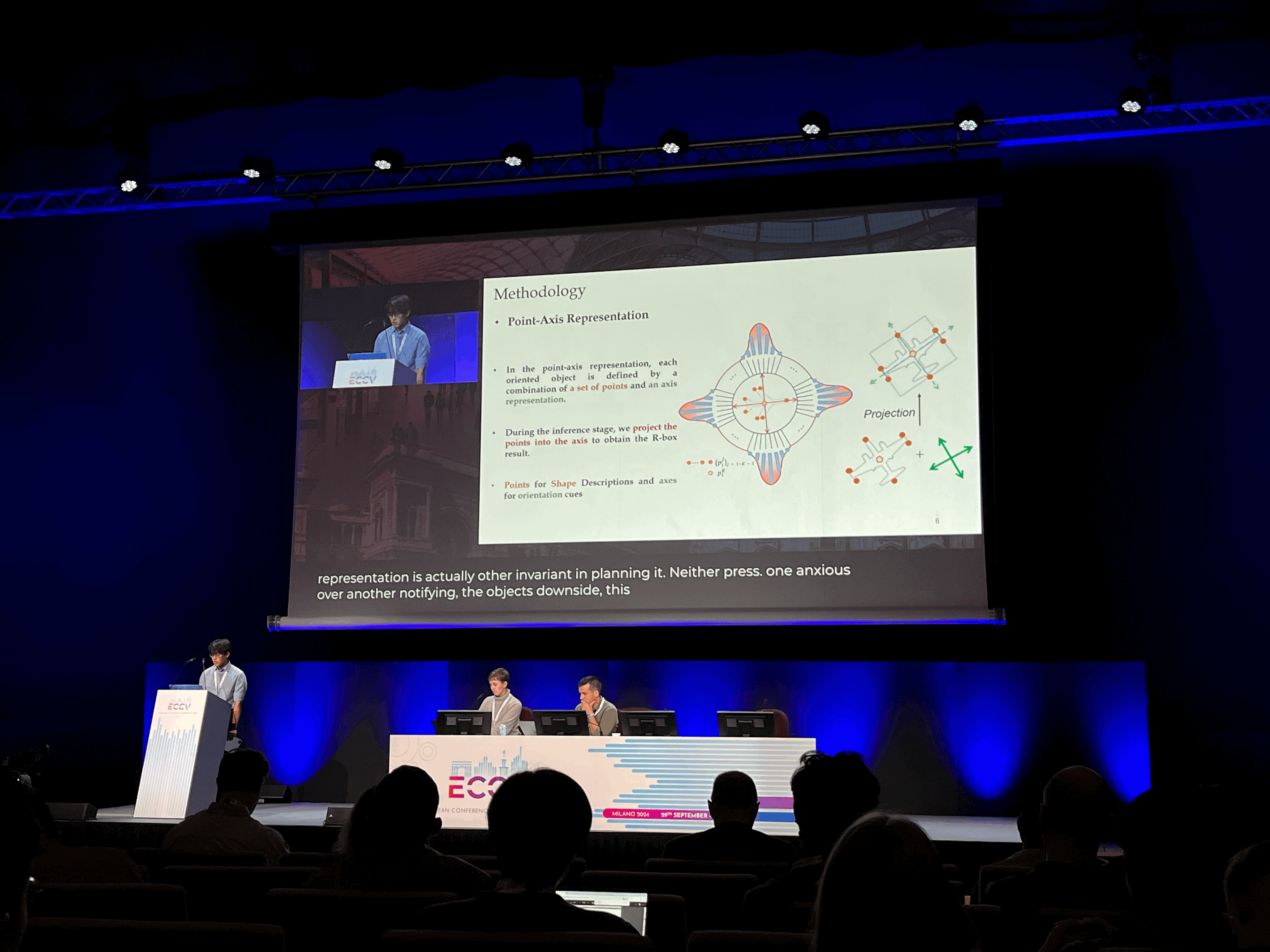
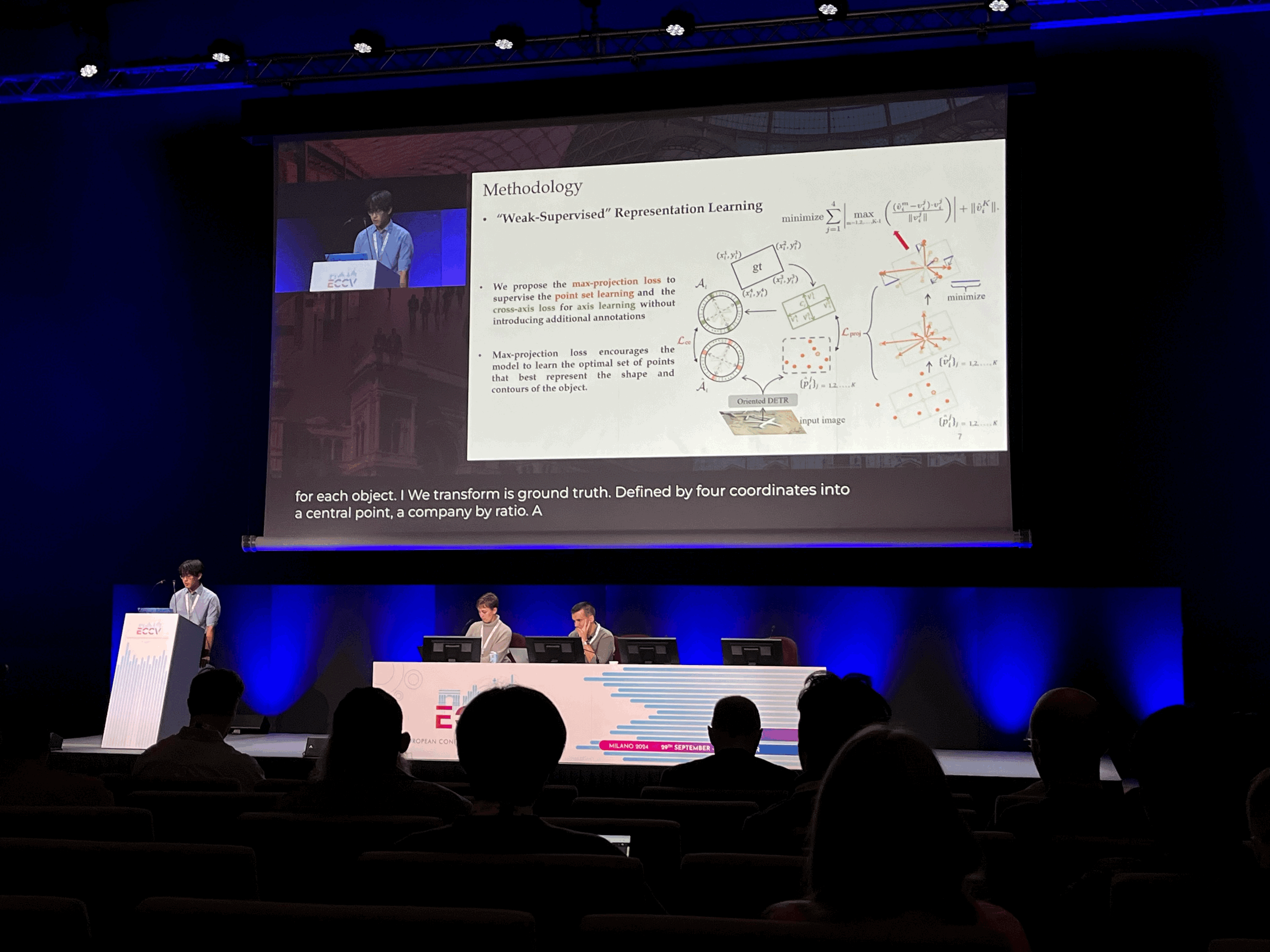
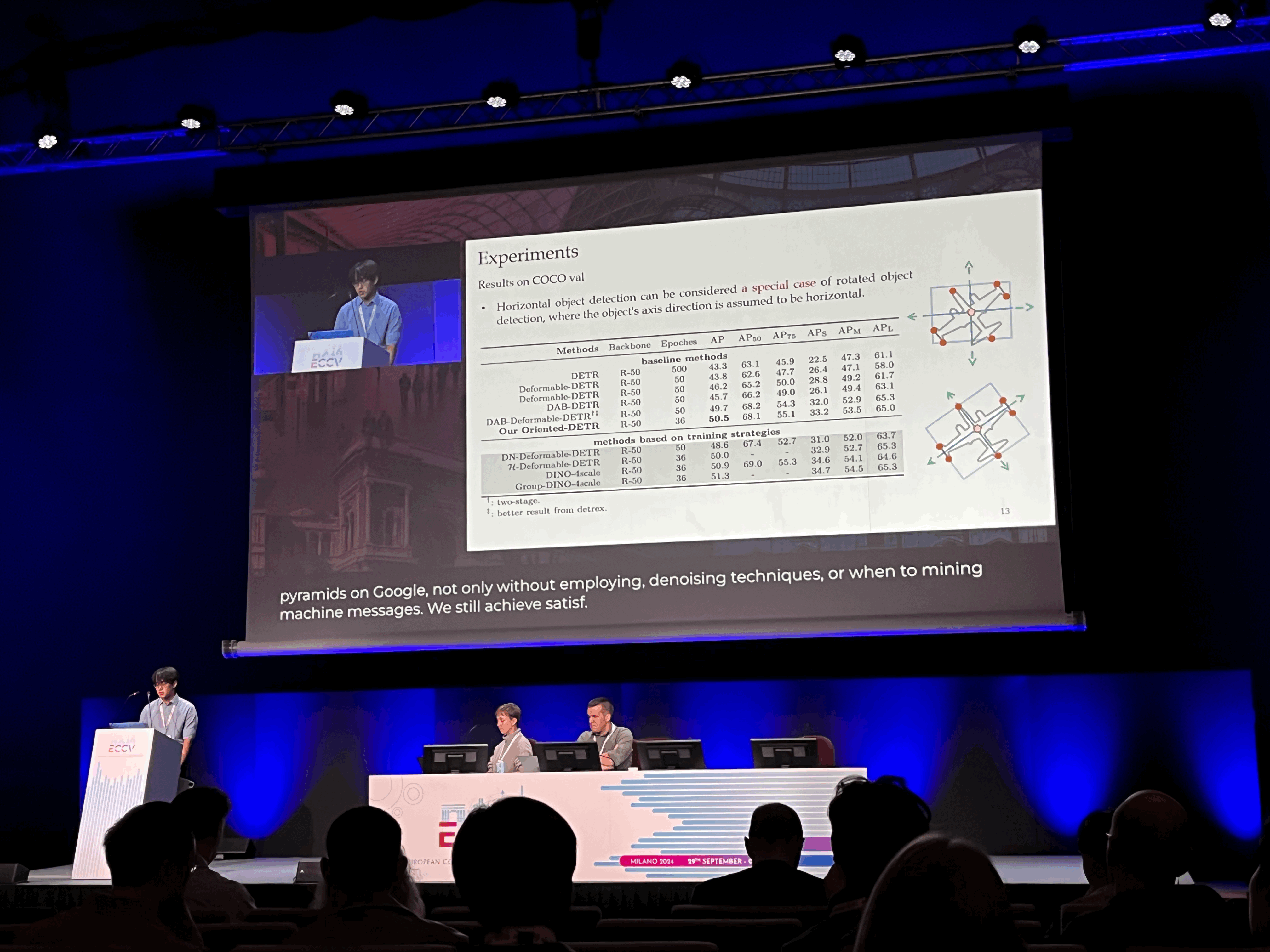
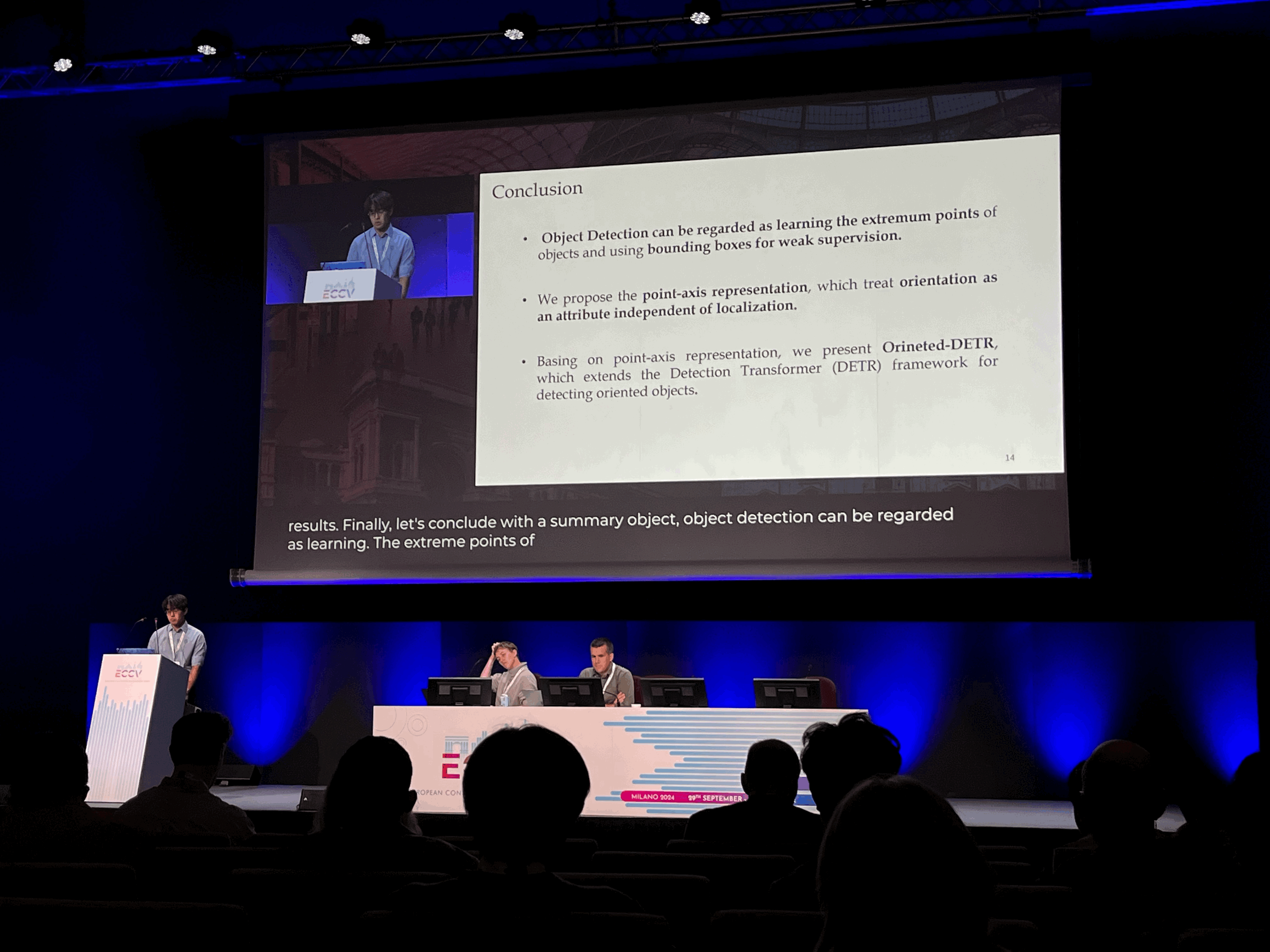
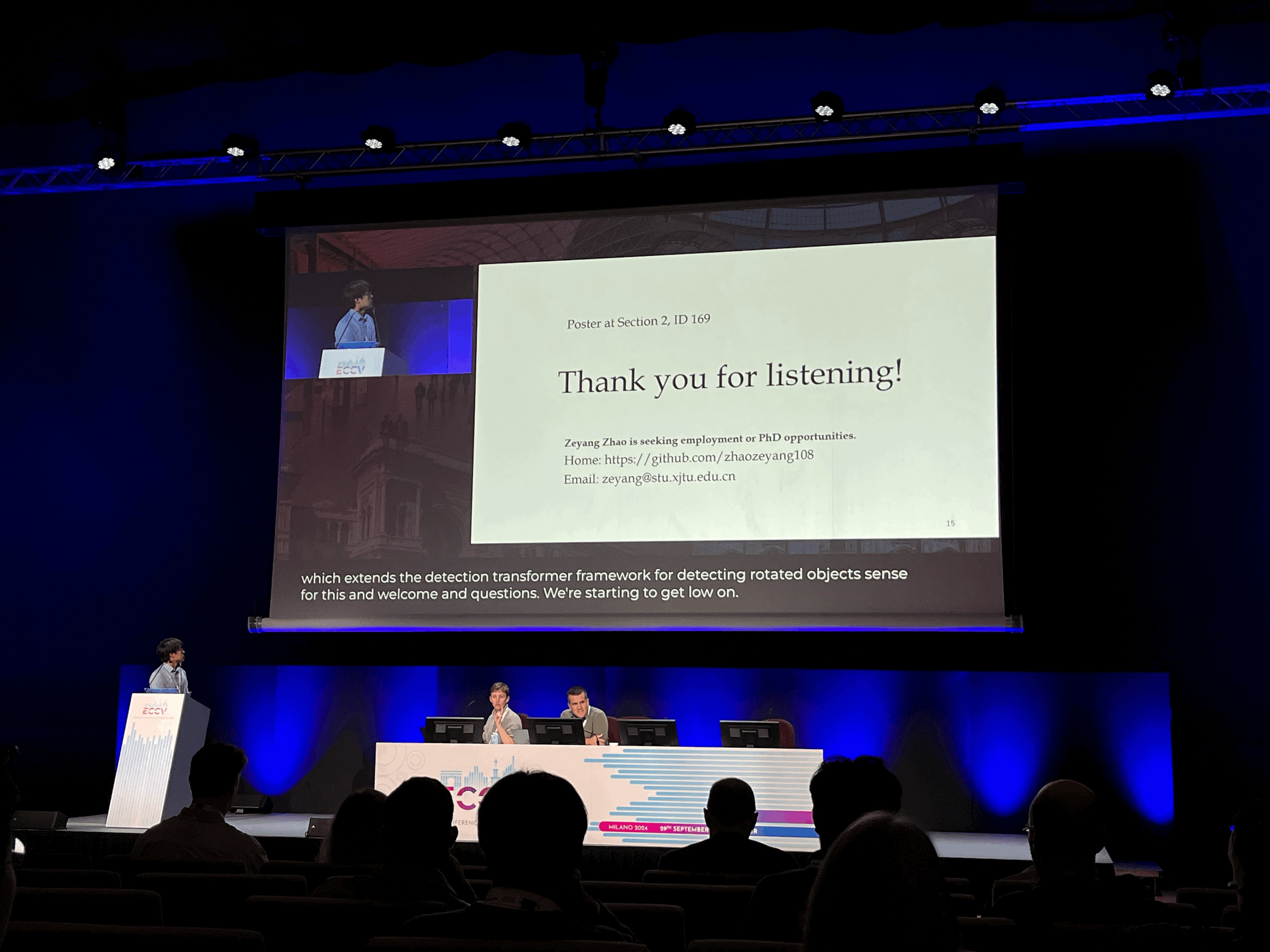
Projecting Points to Axes: Oriented Object Detection via Point-Axis Representation
https://www.ecva.net/papers/eccv_2024/papers_ECCV/papers/04051.pdf
๋ค๋ฅธ ์ต๊ธ์ ์ด๋ป๊ฒ ํํํ ๊ฒ์ธ๊ฐ?์ ๋ํ ์์ด๋์ด๊ฐ ํฅ๋ฏธ๋ก์ ๋ค.
๊ฐ์ฒด์ ํค ํฌ์ธํธ๋ค์ ์์๋ด๋ ๊ฒ -> object detection
X, y๋ฅผ d๋ก ๋ฐ๊พผ๋ค -> direction information
Abstract.
This paper introduces the point-axis representation for oriented object detection, as depicted in aerial images in Figure 1, emphasizing its flexibility and geometrically intuitive nature with two key components: points and axes. 1) Points delineate the spatial extent and contours of objects, providing detailed shape descriptions. 2) Axes define the primary directionalities of objects, providing essential orientation cues crucial for precise detection. The point-axis representation decouples location and rotation, addressing the loss discontinuity issues commonly encountered in traditional bounding box-based approaches.
For effective optimization without introducing additional annotations, we propose the max-projection loss to supervise point set learning and the cross-axis loss for robust axis representation learning. Further, leveraging this representation, we present the Oriented DETR model, seamlessly integrating the DETR framework for precise point-axis prediction and end-to-end detection. Experimental results demonstrate significant performance improvements in oriented object detection tasks.
CLIFF: Continual Latent Diffusion for Open-Vocabulary Object Detection
https://eccv.ecva.net/virtual/2024/oral/488
- ์๊น ๋ฐ๊ฒฝ๋ฌธ ๊ต์๋ ์ฐ๊ตฌ๋ ๊ทธ๋ ๊ณ , Continual learning์ ๋ํด์ ๊ณต๋ถํด๋ณด๋ฉด, ๋ ์ดํด๊ฐ ์ ๋์์ ๊ฒ ๊ฐ๋ค.
Abstract. Open-vocabulary object detection (OVD) utilizes image-level cues to expand the linguistic space of region proposals, thereby facilitating the detection of diverse novel classes. Recent works adapt CLIP embedding by minimizing the object-image and object-text discrepancy combinatorially in a discriminative paradigm. However, they ignore the underlying distribution and the disagreement between the image and text objective, leading to the misaligned distribution between the vision and language sub-space. To address the deficiency, we explore the advanced generative paradigm with distribution perception and propose a novel framework based on the diffusion model, coined Continual Latent Diffusion (CLIFF), which formulates a continual distribution transfer among the object, image, and text latent space probabilistically. CLIFF consists of a Variational Latent Sampler (VLS) enabling the probabilistic modeling and a Continual Diffusion Module (CDM) for the distribution transfer. Specifically, in VLS, we first establish a probabilistic object space with region proposals by estimating distribution parameters. Then, the object-centric noise is sampled from the estimated distribution to generate text embedding for OVD. To achieve this generation process, CDM con- ducts a short-distance object-to-image diffusion from the sampled noise to generate image embedding as the medium, which guides the long-distance diffusion to generate text embedding. Extensive experiments verify that CLIFF can significantly surpass state-of-the-art methods on benchmarks. The code is available at https://github.com/CUHK-AIM-Group/CLIFF.
https://github.com/CUHK-AIM-Group/CLIFF
On Calibration of Object Detectors: Pitfalls, Evaluation and Baselines
Abstract. Reliable usage of object detectors require them to be calibrated - a crucial problem that requires careful attention. Recent approaches to- wards this involve (1) designing new loss functions to obtain calibrated detectors by training them from scratch, and (2) post-hoc Temperature Scaling (TS) that learns to scale the likelihood of a trained detector to output calibrated predictions. These approaches are then evaluated based
on a combination of Detection Expected Calibration Error (D-ECE) and Average Precision. In this work, via extensive analysis and insights, we highlight that these recent evaluation frameworks, evaluation metrics, and the use of TS have notable drawbacks leading to incorrect conclu- sions. As a step towards fixing these issues, we propose a principled evaluation framework to jointly measure calibration and accuracy of object detectors. We also tailor efficient and easy-to-use post-hoc calibration approaches such as Platt Scaling and Isotonic Regression specifically for object detection task. Contrary to the common notion, our experiments show that once designed and evaluated properly, post-hoc calibrators, which are extremely cheap to build and use, are much more powerful and effective than the recent train-time calibration methods. To illustrate, D-DETR with our post-hoc Isotonic Regression calibrator outperforms the recent train-time state-of-the-art calibration method Cal-DETR by more than 7 D-ECE on the COCO dataset. Additionally, we propose improved versions of the recently proposed Localization-aware ECE and show the efficacy of our method on these metrics. Code is available at: https://github.com/fiveai/detection_calibration.
https://github.com/fiveai/detection_calibration
์ํฉํธ ์๋ ๋ฐํ์๋ฃ๋ฅผ ๋ง๋ค๋ ค๋ฉด ๊ตต์ ๋ผ์ธ์ ์ฌ์ฉํ์
์ฝ๊ฐ์ ์ ๋๋ฉ์ด์ ์ผ๋ก ๋ชฐ์ ์ํค๊ธฐ (๊ทธ๋ํ์์ ์ฎ๊ฒจ๊ฐ๊ธฐ)
ํฐ ๋ชฉ์๋ฆฌ ยท ์์ ๊ฐ ์๋ ์์ธ
์ฌ์ฉํ๊ธฐ ์ฌ์์ ๊ฐ์กฐํ์ จ๋ค
'ArtificialIntelligence > ECCV2024' ์นดํ ๊ณ ๋ฆฌ์ ๋ค๋ฅธ ๊ธ
ECCV 2024 Day3 - SAM2: Meta Technical Presentation (0) | 2024.11.18 |
---|---|
ECCV 2024 Day3 - Synthesia Keynote (2) | 2024.11.14 |
ECCV 2024 DAY3 - Demo Session (7) | 2024.10.18 |
ECCV 2024 DAY2 - Dataset Distillation Workshop (2) (2) | 2024.10.08 |
ECCV 2024 DAY2 - Dataset Distillation Workshop (1) (0) | 2024.10.08 |