2024. 11. 18. 21:20ใArtificialIntelligence/ECCV2024
ECCV 2024. 10. 02. Wednesday
Oral 3A: Datasets And Benchmarking
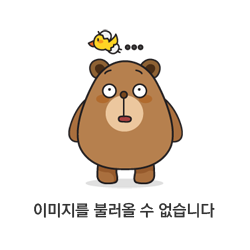
์ค๋์ ๊ผญ ๋ค์ด์ผ ํ ์ฃผ์ ๊ฐ ์๊ธฐ ๋๋ฌธ์,
์ค์ ๋ฐํ๋ฅผ ๋ฃ๊ธฐ ์ํด, ์ผ์ฐ ๋์ฐ์ต๋๋ค โ๏ธ
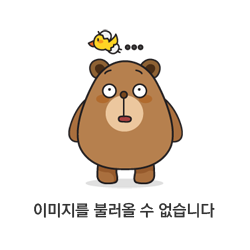
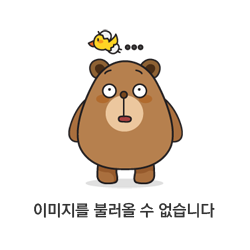
Oral: Dataset Condensation
Towards Model-Agnostic Dataset Condensation by Heterogeneous Models
Jun-Yeong Moon ยท Jung Uk Kim ยท Gyeong-Moon Park
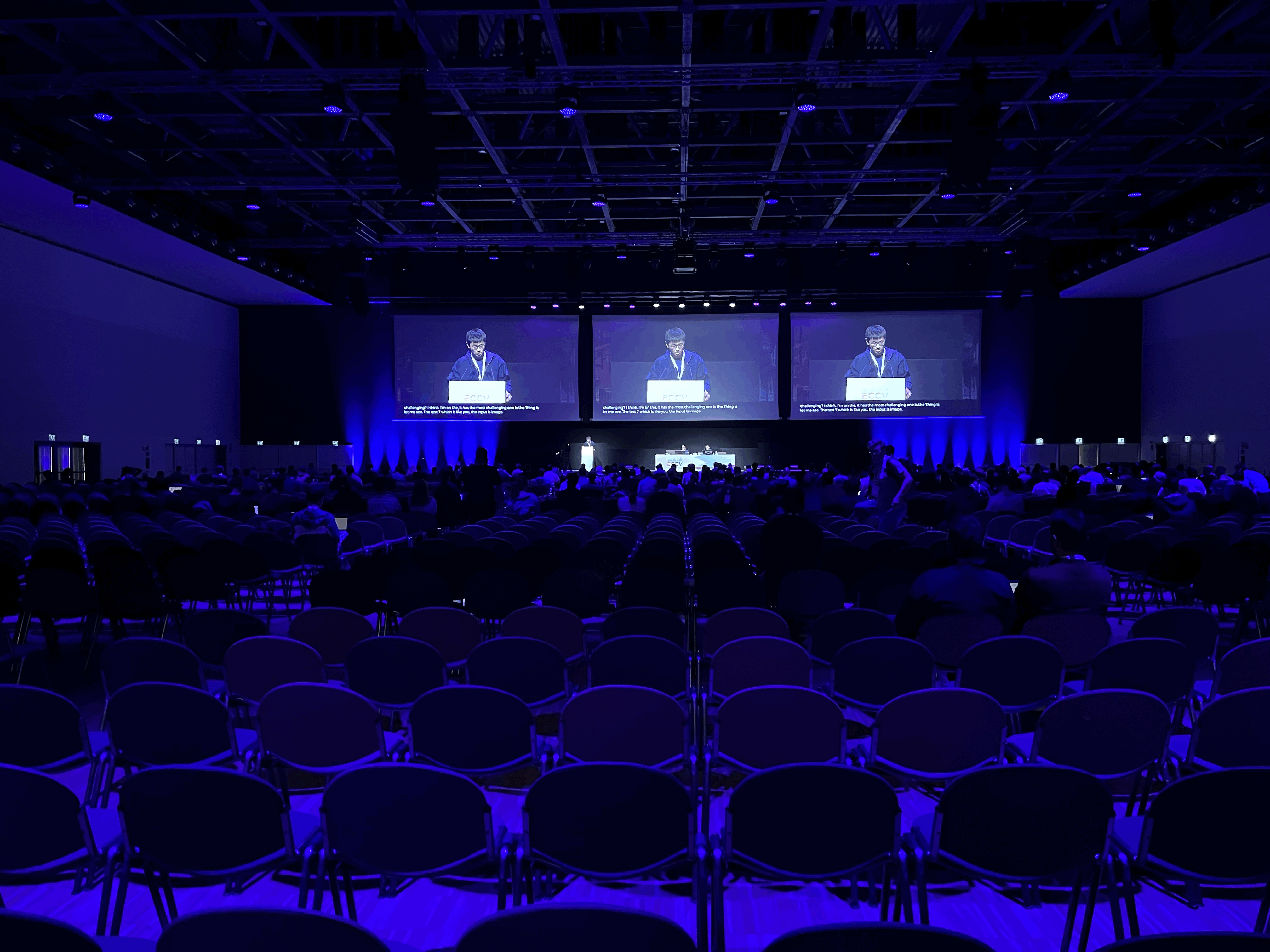
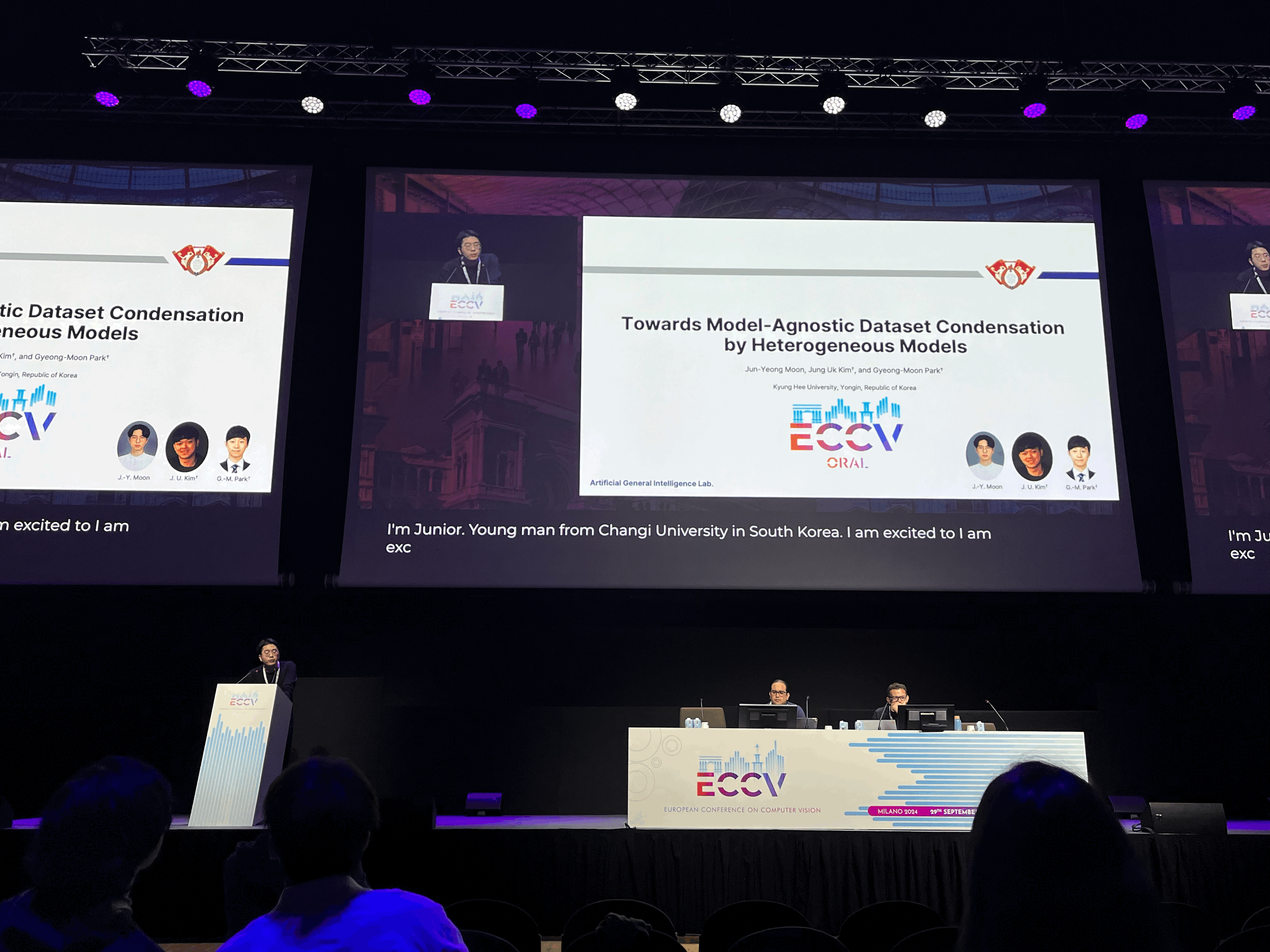
https://eccv.ecva.net/virtual/2024/oral/856
ECCV 2024 Towards Model-Agnostic Dataset Condensation by Heterogeneous Models Oral
Registration Required You must be logged in to view this content.
eccv.ecva.net
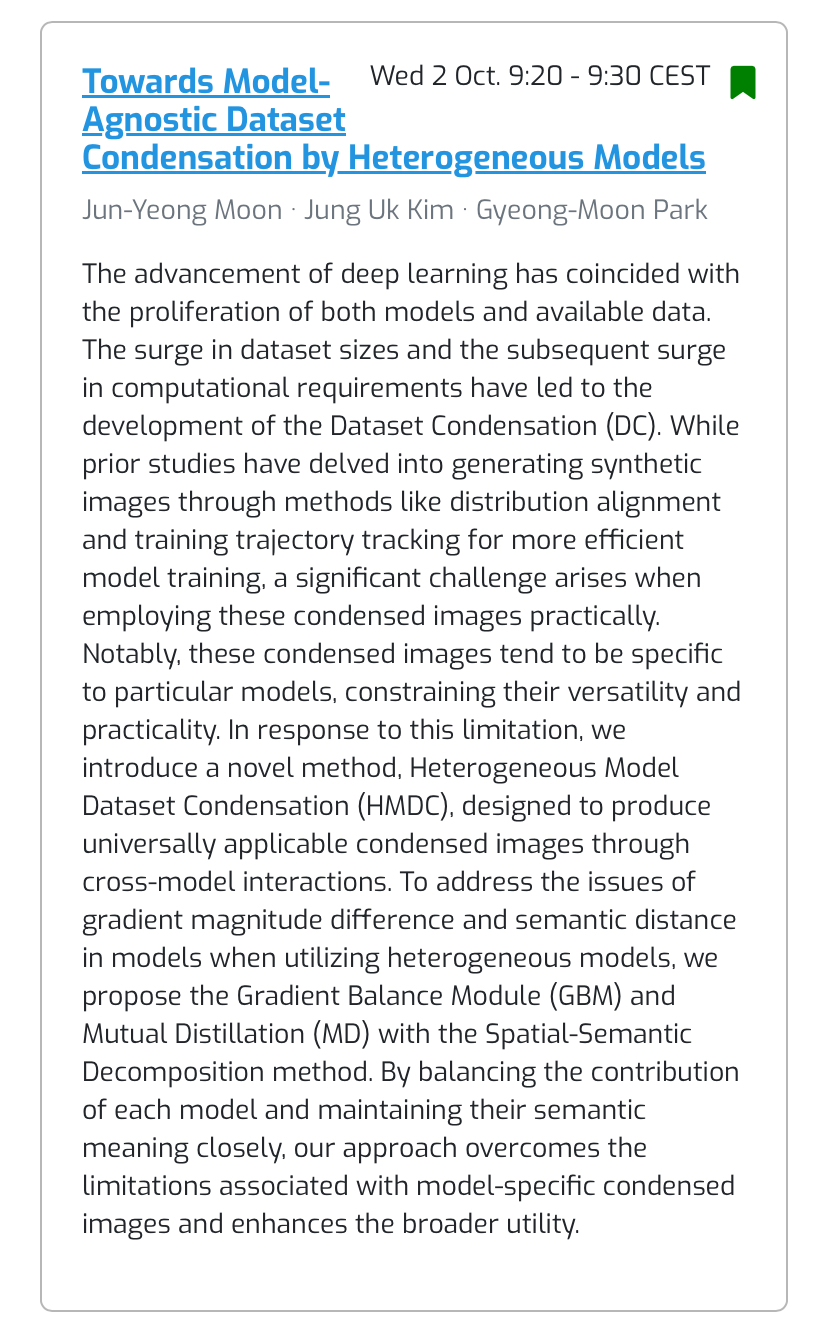
Abstract
The advancement of deep learning has coincided with the proliferation of both models and available data. The surge in dataset sizes and the subsequent surge in computational requirements have led to the development of the Dataset Condensation (DC). While prior studies have delved into generating synthetic images through methods like distribution alignment and training trajectory tracking for more efficient model training, a significant challenge arises when employing these condensed images practically. Notably, these condensed images tend to be specific to particular models, constraining their versatility and practicality.
In response to this limitation, we introduce a novel method, Heterogeneous Model Dataset Condensation (HMDC), designed to produce universally applicable condensed images through cross-model interactions. To address the issues of gradient magnitude difference and semantic distance in models when utilizing heterogeneous models, we propose the Gradient Balance Module (GBM) and Mutual Distillation (MD) with the Spatial-Semantic Decomposition method. By balancing the contribution of each model and maintaining their semantic meaning closely, our approach overcomes the limitations associated with model-specific condensed images and enhances the broader utility.
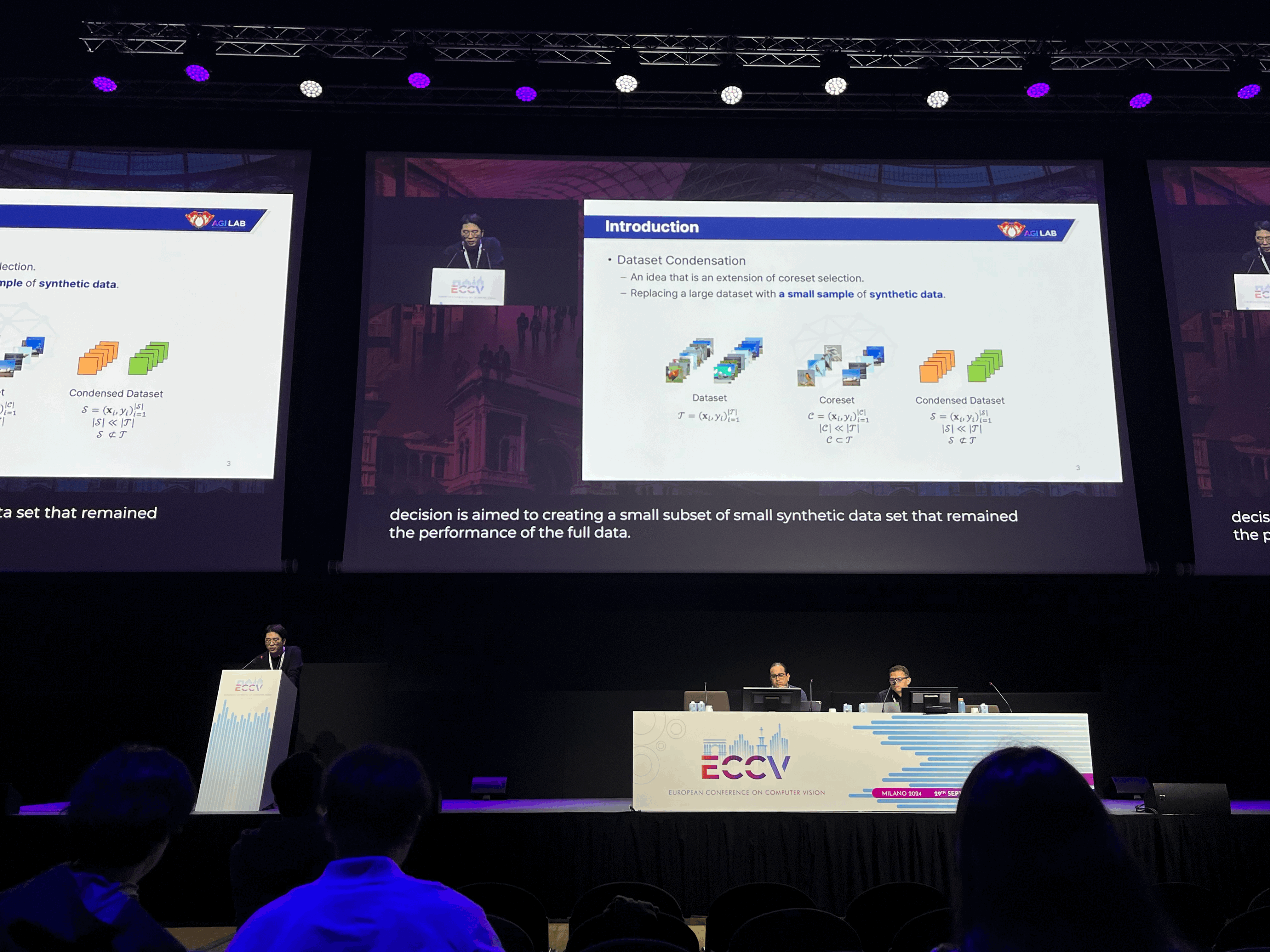
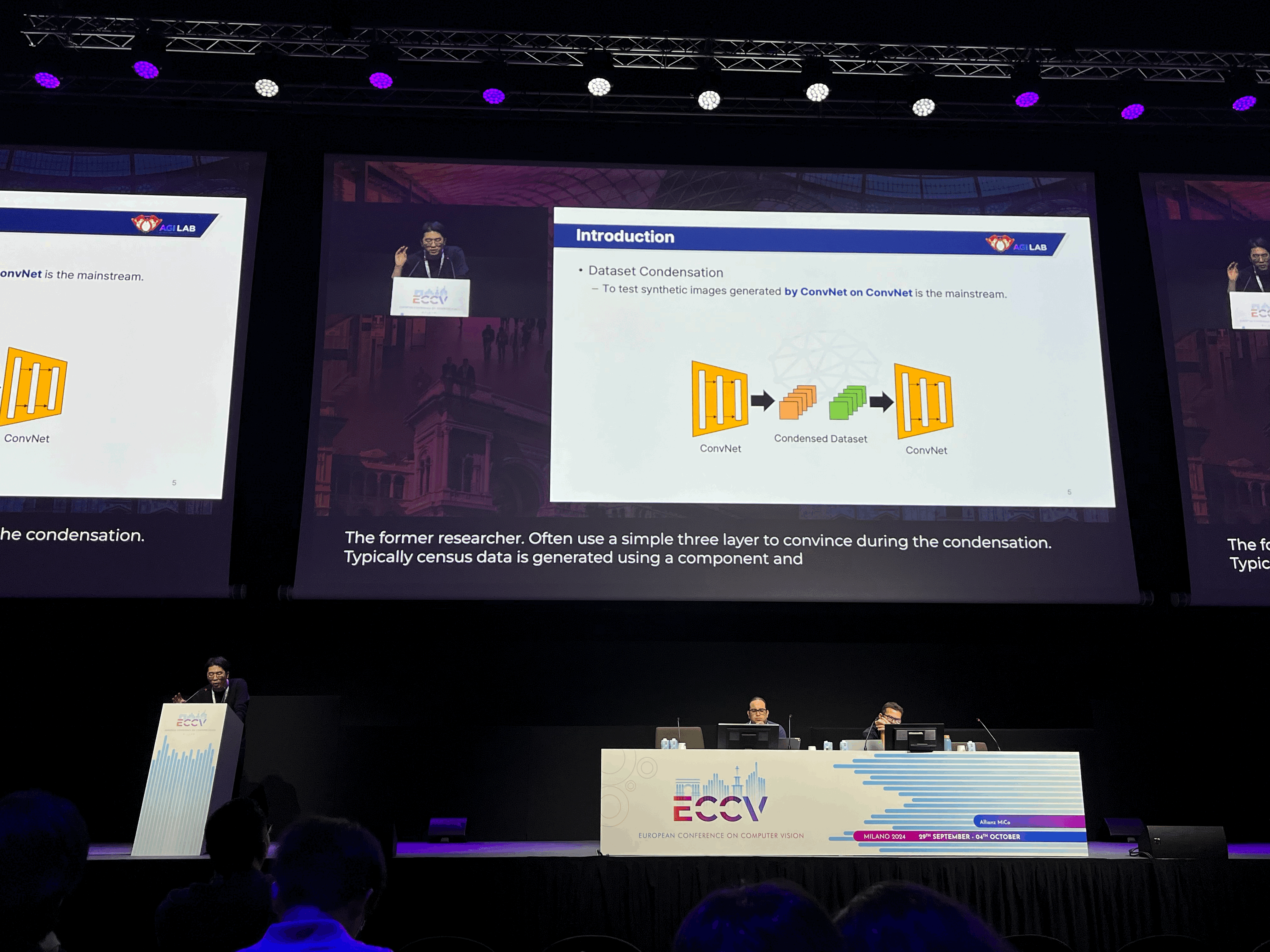
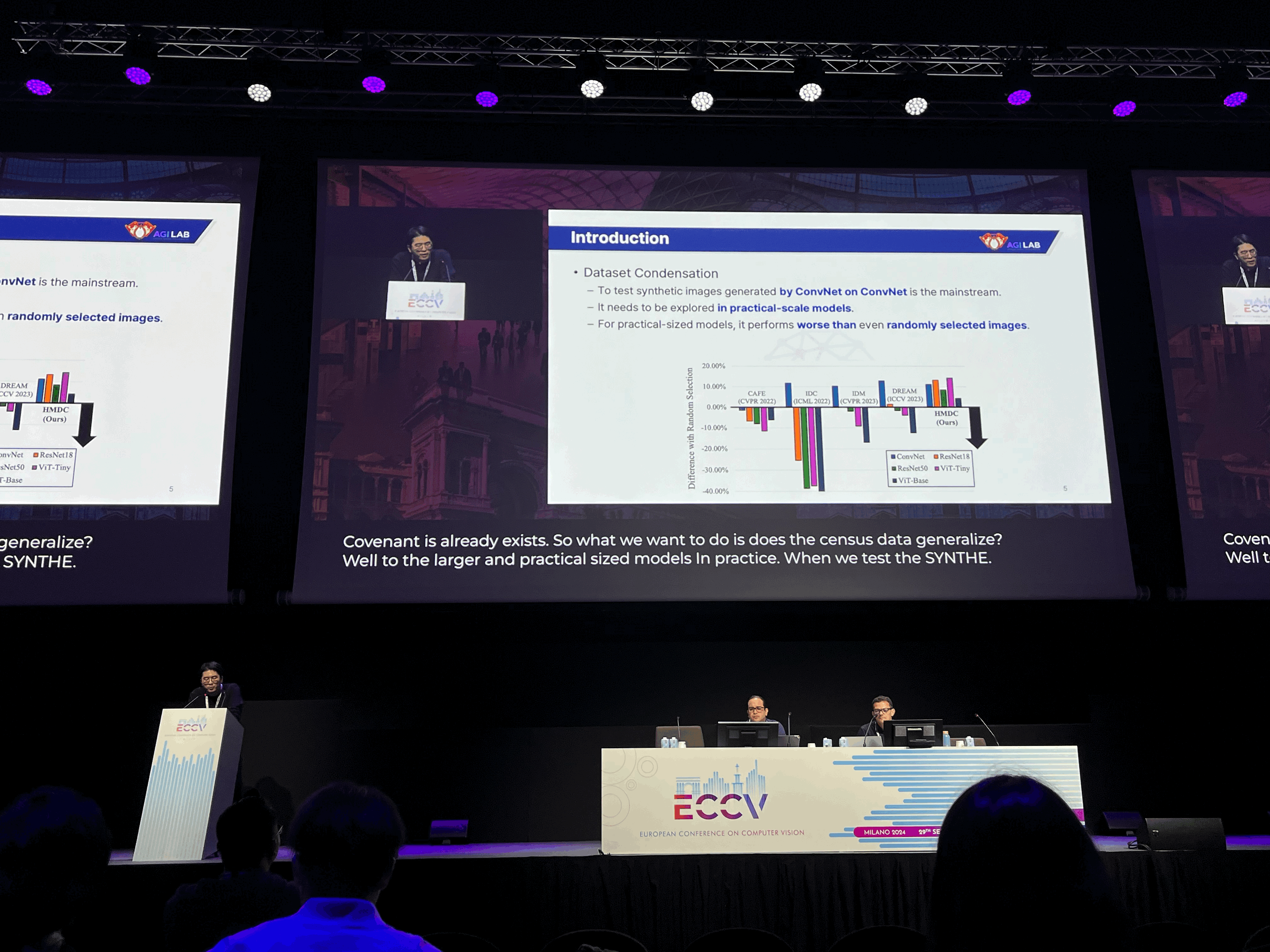
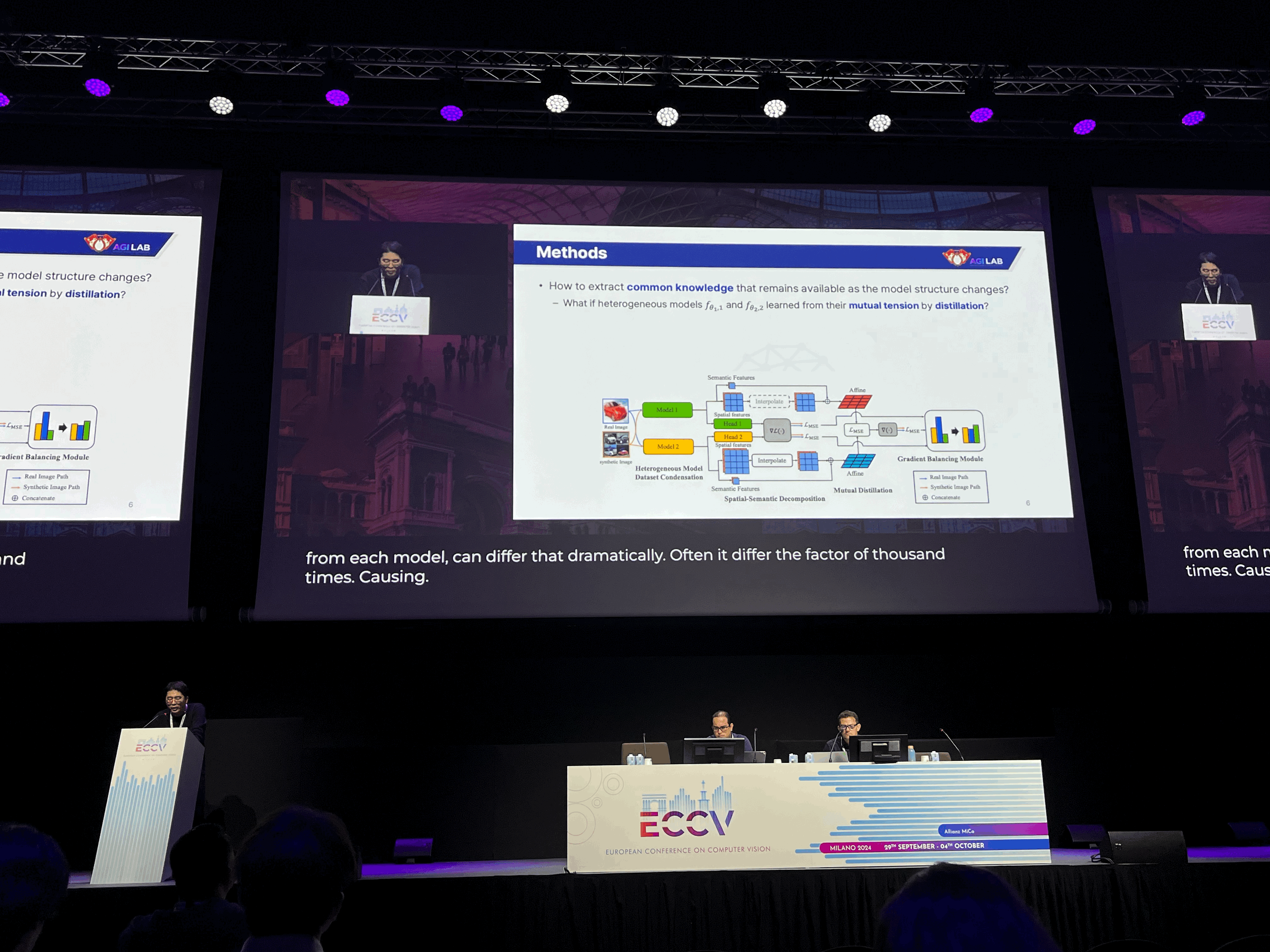
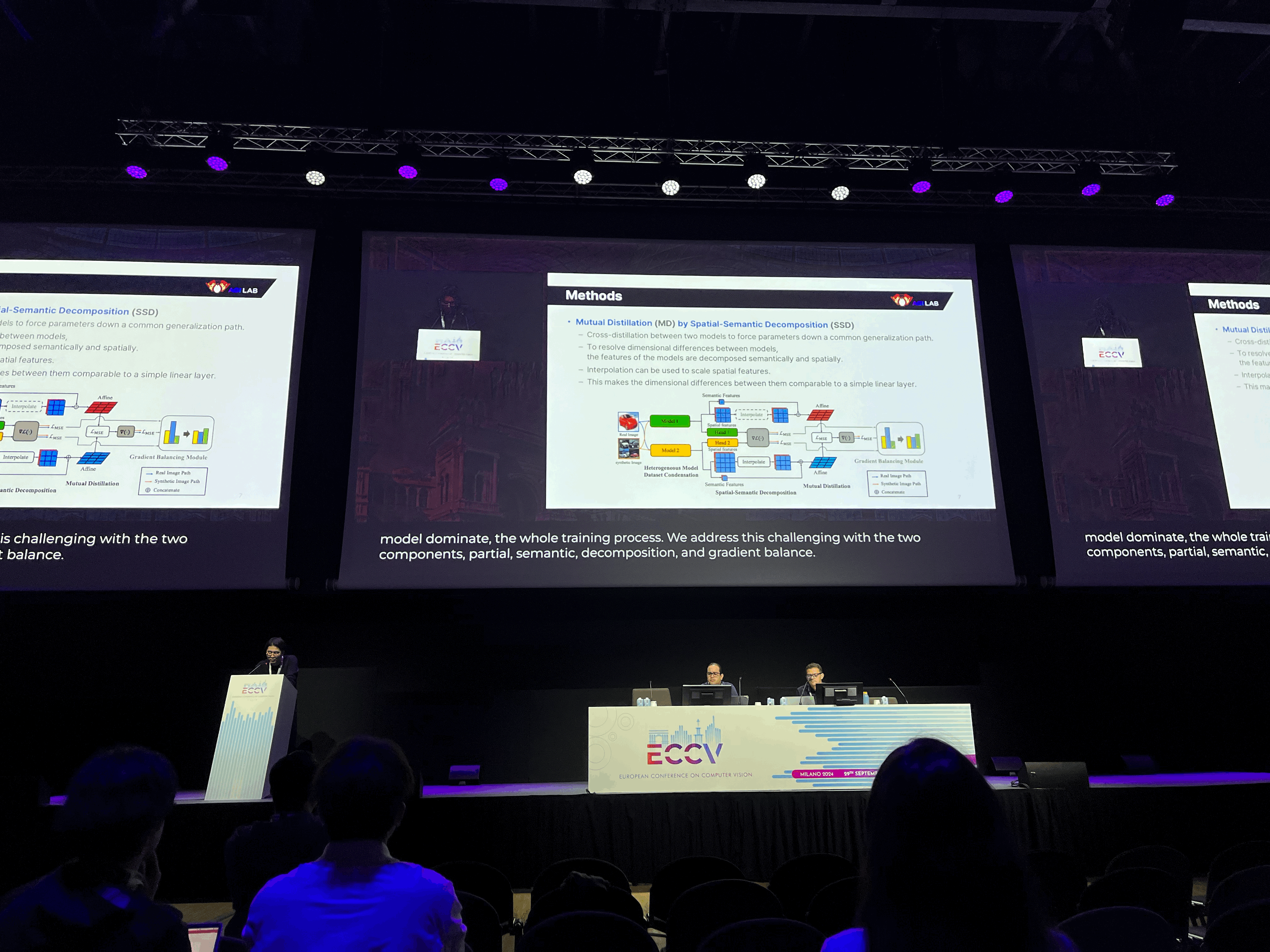
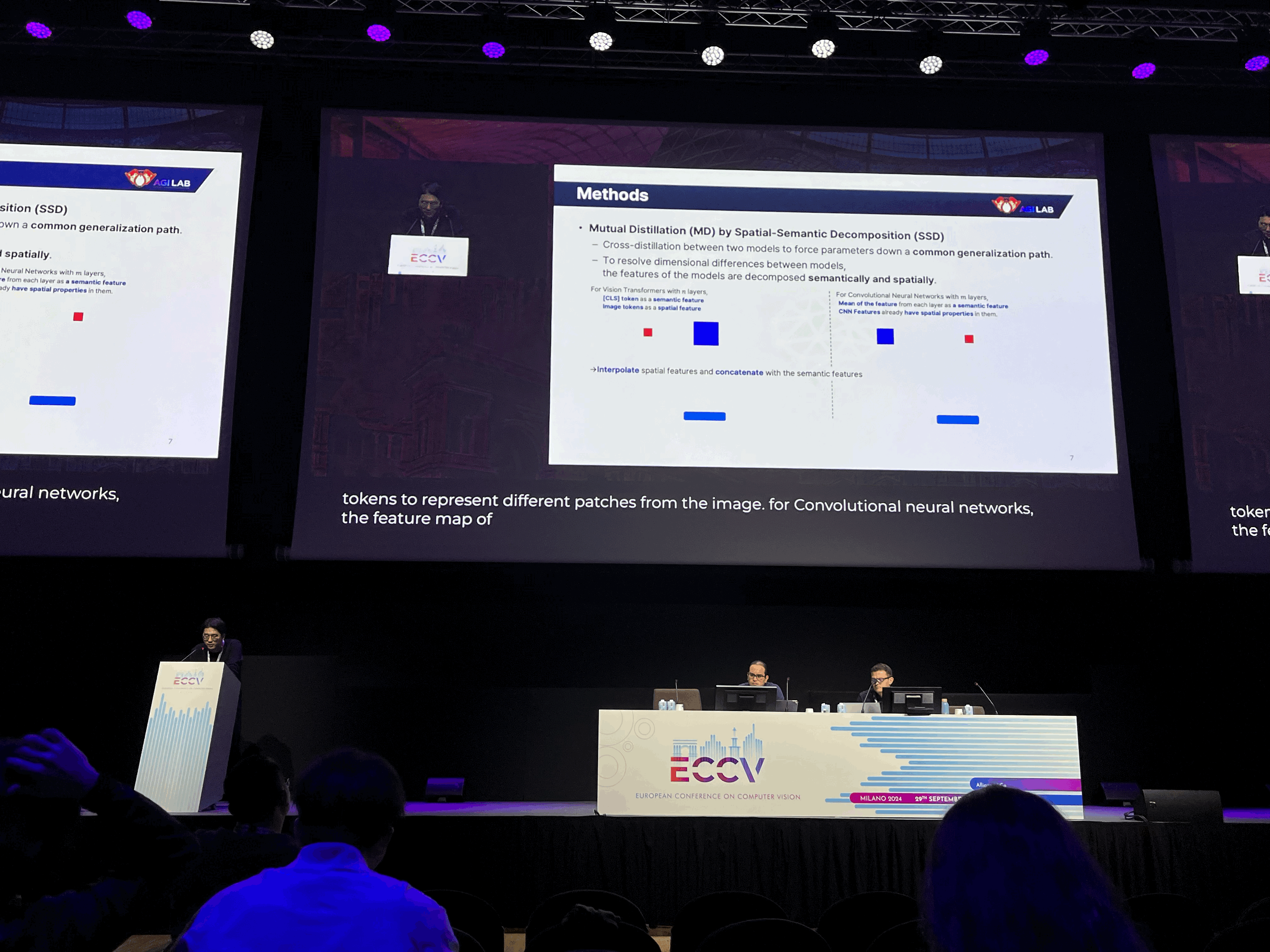
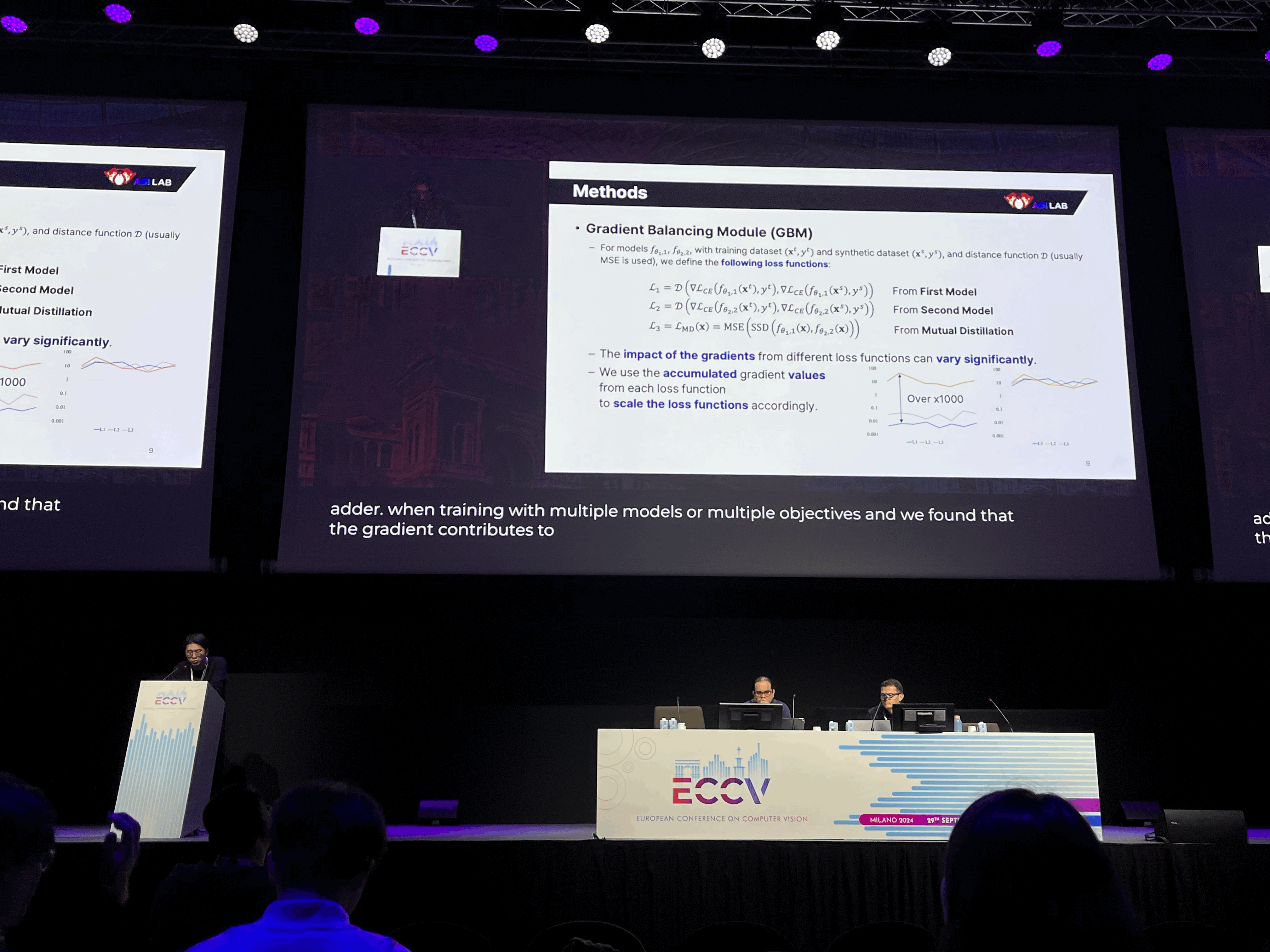
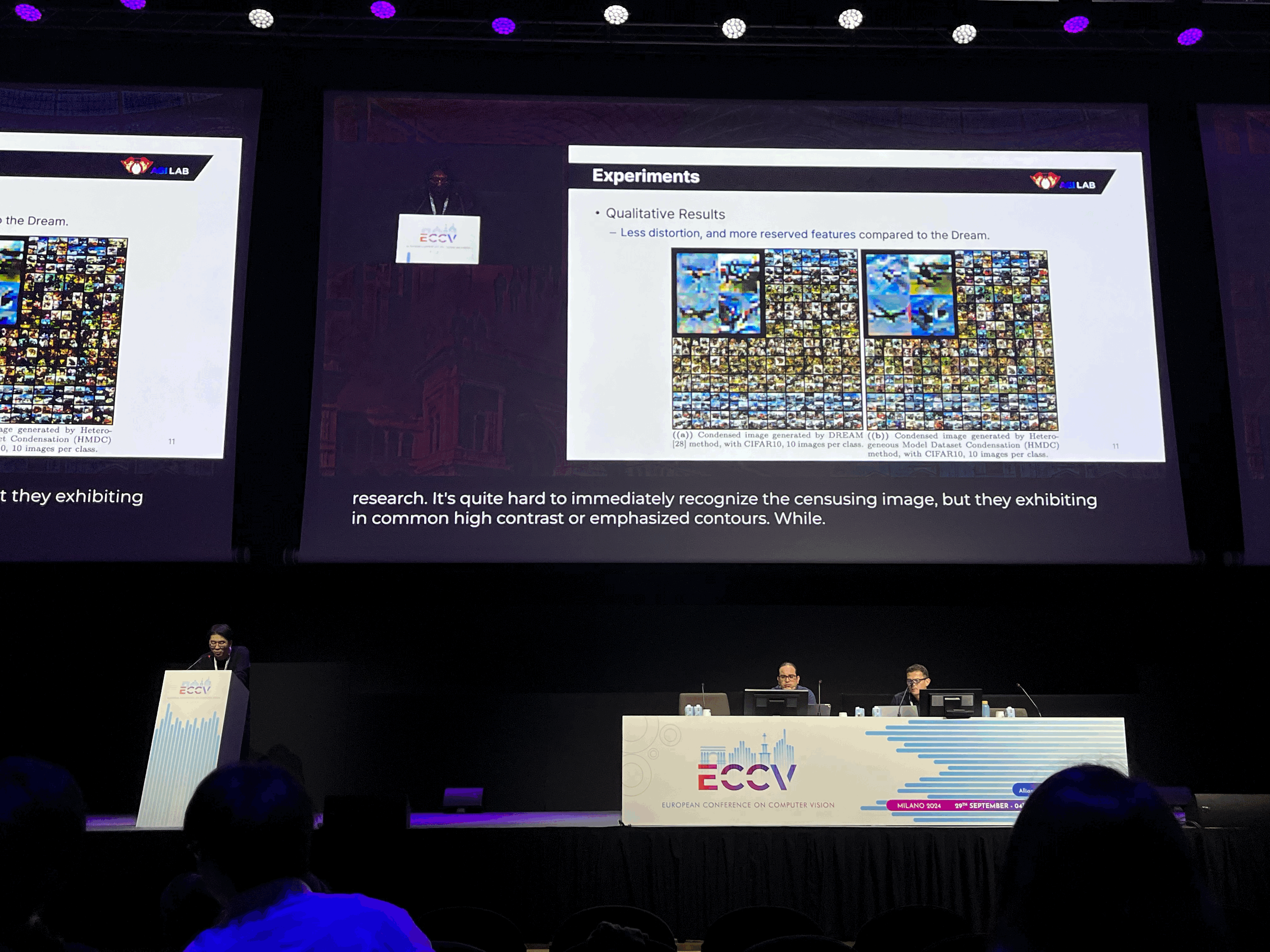
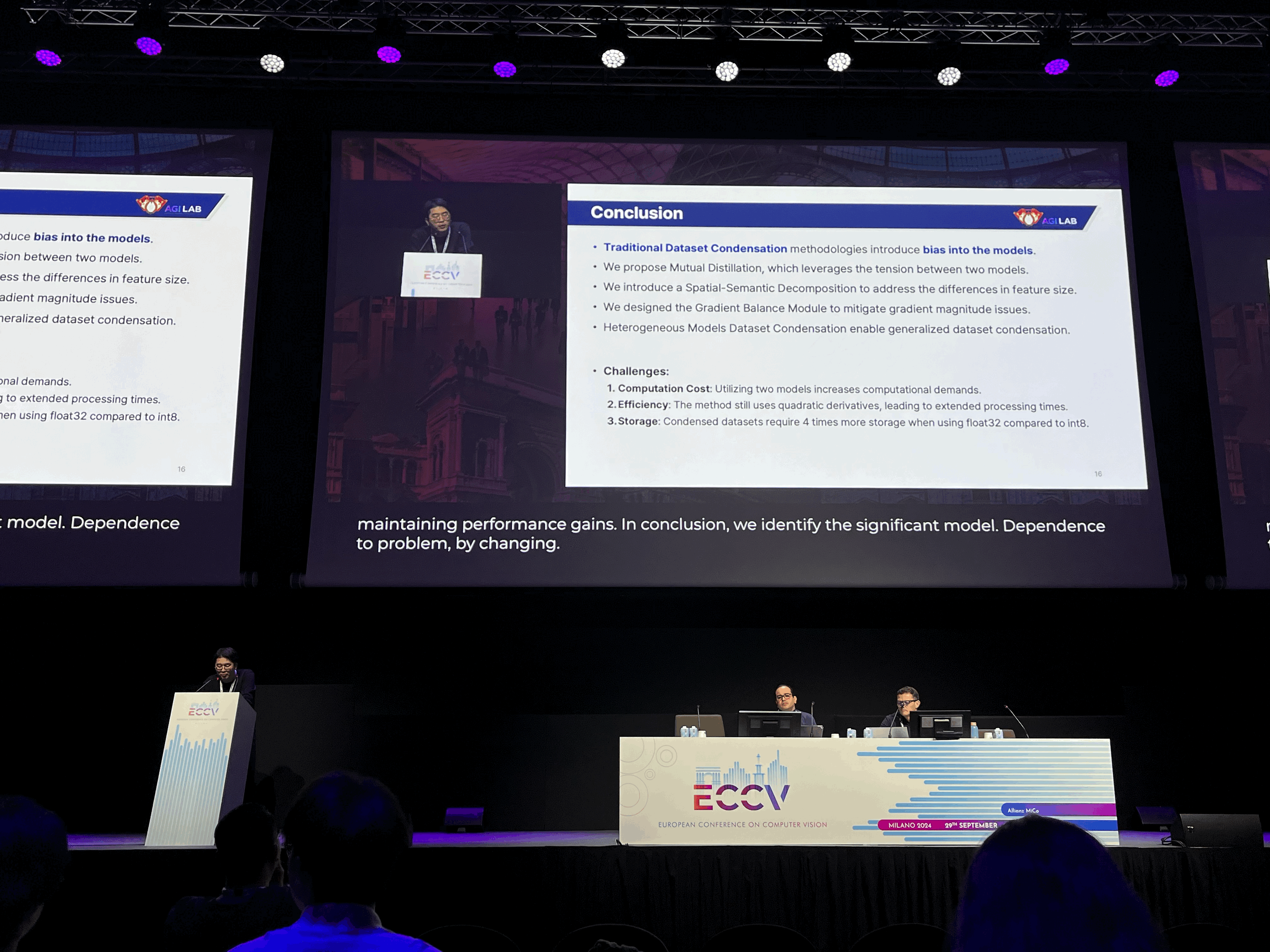
๐ Git-hub Link
https://github.com/KHU-AGI/HMDC
GitHub - KHU-AGI/HMDC: Official Repository for Heterogeneous Models Dataset Condensation (ECCV 2024, Oral)
Official Repository for Heterogeneous Models Dataset Condensation (ECCV 2024, Oral) - KHU-AGI/HMDC
github.com
'ArtificialIntelligence > ECCV2024' ์นดํ ๊ณ ๋ฆฌ์ ๋ค๋ฅธ ๊ธ
ECCV 2024 Day4 - Oral: Point Clouds (1) | 2024.11.18 |
---|---|
ECCV 2024 Day3 - SAM2: Meta Technical Presentation (0) | 2024.11.18 |
ECCV 2024 Day3 - Synthesia Keynote (2) | 2024.11.14 |
ECCV 2024 Day3 - Oral: Recognition (0) | 2024.11.13 |
ECCV 2024 DAY3 - Demo Session (7) | 2024.10.18 |